Analysis. A conversation with Arun Gururajan, NetApp’s VP for research and data science and a GenAI expert, has shed light on the use of vector embeddings in chatbots for leveraging an organization’s proprietary textual data.
I had previously learned from DataStax and Barnacle.ai founder John-David Wuarin that this would be a time-consuming and computationally intensive task. Gururajan suggests that it is less time and compute-intensive than we might think. But there are nuances to bear in mind.
Let’s just set the scene here. Generative AI involves machine learning large language models (LLMs) being given a request. They operate on stored data and mount a semantic search of it to find items that correspond as much as possible to what it is looking for. The LLMs don’t understand – in a human sense – what they are looking for. They turn a request into vector embeddings – code strings or hashes for multiple dimensions of the request, then search a vector database for similar vector embeddings.
There isn’t a one-to-one relationship between words and vectors. Vectors will be calculated for tokens, parts of words, whole words or groups of words.
An organization will generally want the LLMs it uses to search its own proprietary data – retrieval-augmented generation (RAG) – to find the most relevant responses to employee requests. But that means the proprietary data needs vectorizing before it can be semantically searched. This background can be explored further here.
The data estate could involve, text, images, audio, and video files. The first pass general LLM usage will focus on text and that’s what we look at here.
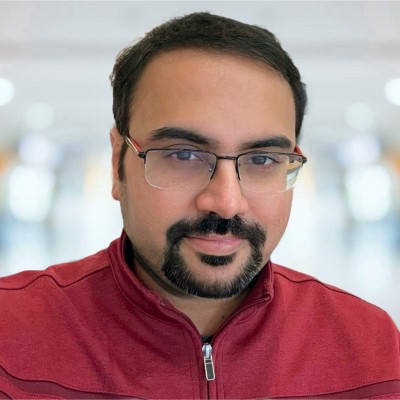
Gururajan and I talked in a briefing last week and he explained: “I come from Meta. I was leading applied research at Meta, where I was building all things from like multimodal deep learning models for fraud detection on Facebook, and Instagram, building all the search and personalization for Meta’s online store. And of course, solving their supply chain problems doing traditional optimization problems as well.
“Before that, I spent a while at Microsoft, where I led multiple teams on AI working on cyber security for Microsoft 365. And my Master’s and PhD were in computer vision. So I’ve been at this for about a couple of decades now.”
He made the point that an organization’s text-based data estate might not be as large as all that. “If you take Wikipedia, for example, which is a significant corpus of text representing most of the world’s knowledge, the textual form of Wikipedia is less than 40 gigabytes.”
That’s a surprise. I was thinking it amounted to tens of terabytes. Not so.
He noted my surprise: “A lot of people will give me the surprised look when I tell them, but if you take Wikipedia as just textual articles, it is less than 40 gigabytes … So most organizations, I would say 80 percent of the organizations, will have textual data that’s probably less than that.”
Pre-processing
You can’t just give this raw text data to an LLM. There will be pre-processing time. Gururajan continued: “Data can be in Word documents, it could be in PDF documents, it could be in HTML. So you need to have a parser that takes all those documents and extracts that text out. Once you parse out the text, you need to normalize the data. Normalization is like you might want to remove punctuation, you might want to remove capitalization, all of those things. And then you want to tokenize the words.”
Documents might need converting into sub-chunks. “For example, a HR document might contain something about finance, it may contain some other topics. Typically, you want to chunk the documents [and] the chunking process is also part of the pre-processing … The pre-processing does take a finite amount of time.”
Now we come to the next aspect of all this. “The third thing is the complexity of the embedding model. Because you need to take all those document chunks, and you want to convert that into a vector, and that’s typically done by an embedding model. And embedding models come across in a variety of sizes. If you look at Hugging Face [and its] massive text embedding benchmark leaderboard, you can see a list of embedding models which are on the leaderboard, and categorized by their size.
“You can look at models less than 100 million parameters, between 100 million to 500 million, up to a billion, and greater than a billion. Within each category, you can pick a model. Obviously, there are trade-offs, so if you pick a model with a huge computational footprint, computing the embedding – which means you are doing a forward pass on the model – is going to take more time.”
He explained a lot of the applications use very compact models – such as MiniLM, a distilled version of Google’s Bert model. This is fast and can convert a chunk to a vector in milliiseconds.
Another factor comes into play here. “The fourth thing is dimensionality of the embeddings. If you want the vector to be 380-dimensional? Great. If you want it to be 2,000 dimensional then you’re going to require more computation.”
If you choose a long vector, it means that you’re getting more semantic meaning from the chunk. However, “it really is a trade-off between how much you want to extract versus how much latency you want. Keep in mind that the vector length also impacts the latency the user faces when interacting with the LLM.”
He said lots of people have found a happy medium with the 384-dimensional vector and MiniLM, which works across 100 languages by the way.
Now “the embeddings creation for RAG is mostly like a one time process. So you can actually afford to throw a bunch of pay-as-you-go compute at it, you can throw a GPU cluster at it.” Doing this,”“I can tell you that the all of Wikipedia can be embedded and indexed within 15 minutes.”
The embedding job you send to a GPU farm needs organizing. “If you’re not thoughtful about how you’re batching your data and sending it to the GPU then you’re going to be memory bound and not compute bound, meaning that you’re not going to be using the full computational bandwidth of the GPU,” and wasting money.
Indexing
The next point to bear in mind is the index of the embeddings that an LLM uses. “When the user puts in a new query, that query is converted to a vector. And you cannot compute the similarity of the vector to all of the existing vectors. It’s just not feasible.”
So when selecting a vector database with in-built indexing processes, “you need to be smart about how you are indexing those vectors that you’ve computed … You can choose how you want to index your embeddings, what algorithm that you want to use for indexing your embeddings.”
For example, “there’s K nearest neighbor; that is approximate nearest neighbor.” The choice you make “will impact the indexing time. Keep in mind that vector indexing can also be done on a GPU, so if you’re going to throw compute at for the embedding, you might as well use the compute for indexing as well.”
What this comes down to in terms of time is that “for most enterprises, and I’m just giving a ballpark number, if they really throw high-end GPUs, they are really talking in the order of minutes, tens of minutes for embedding their data. If you’re throwing mid-size GPUs, like A10s or something like that, I would say you’re really talking about an order of a few hours for indexing your data.
“If you’re talking about hundreds of gigabytes of data, I would say it’s probable we are talking about a ten-hour time to indexing your entire data set.”
Up until now, this is all about RAG vectorization and indexing for text. It won’t stop there.
Multi-modal RAG
Gururajan continued: “The next evolution of RAG … is that there are going to be three different embedding models. There’s one embedding model that takes text and converts it to embeddings. There’s going to be another embedding model that either takes video or images and then converts that into embeddings. So those are two embedding models.
“Then there’s a third embedding model which actually takes these two embeddings, the text embeddings and the images beddings and maps it to a common embedding space. And we call this as contrastive learning.”
A V7 blog explains this “enhances the performance of vision tasks by using the principle of contrasting samples against each other to learn attributes that are common between data classes and attributes that set apart a data class from another.”
It adds time, as Gururajan explained: “When you’re thinking about three different embedding models, when you’re doing multimodal RAG, you might end up doubling the time it takes to generate the embeddings or slightly more than that … But it should not really be something that will take the order of days. It will probably be like a day to get everything set.”
An organization could hire a group of people to do this, but Gururajan thinks that won’t be the general pattern. Instead people will use tools, many open source, and do it themselves. He observed: “So many tools have come up that actually abstract out a lot of things. The whole of the field has become democratized.”
DIY or hire bodies?
“You take a tool, and it says these are all the ML algorithms, which ones do you want to run? It actually gives you a default selection. You almost get a Turbo Tax-like interface, you just click click, and you’re done building a model.”
He thinks the RAG area is at an inflexion point, with tools and optimizations appearing. “People are going to create open source and, in fact, they’ve already started coming out, open source RAG frameworks.”
These would “package everything for you, from data loaders to tokenizers to embedding models, and it makes intelligent choices for you. And obviously, they are going to tie it with the GPU compute and make it so that a good engineer should be able to use it end to end.”
We can envisage NetApp possibly making such frameworks available to its customers.
Hallucinations
Can RAG reduce LLM hallucinations? One of the characteristics of an LLM is that it doesn’t know it’s hallucinating. All it knows is what’s likely to follow a particular word in a string of text, based on probabilities. And the probabilities could be high, or they could be low, but they still exist. In Gururajan’s view: “LLMs are autoregressive machines, meaning they essentially look at the previous word, and then they try to predict the next one; they create a probability distribution and predict the next word. So that’s all there is to an LLM.
“There’s a lot of research going on in knowledge graphs that can augment this retrieval process so that the LLM can summarize it in a better manner.” The LLM uses a recursive chain of reasoning. “You have a chain of prompts that make the LLM look at the article again, and again, make it look at the document from different angles with very targeted prompts.”
This can help reduce hallucinations, but it’s not a cure. Gururajan asks: “Can we completely reduce hallucinations? No, I don’t think there’s any framework in the world that’s there yet that can claim zero hallucinations. And if something claims zero hallucinations, I would really be very wary of that claim.”