Assured Data Protection, the largest global Managed Service Provider (MSP) for Rubrik, has launched in the Middle East offering fully managed backup and cyber recovery services to businesses of all sizes. Assured has been rapidly growing its footprint in 2024 following successful launches in Canada and Latin America. This Middle East expansion is supported by a strategic partnership with Mindware, a leading Value-Added Distributor (VAD) in the region. The collaboration will establish local datacenters to help clients manage data sovereignty issues and minimize latency in data transfer, enhancing operational efficiency and security.
…
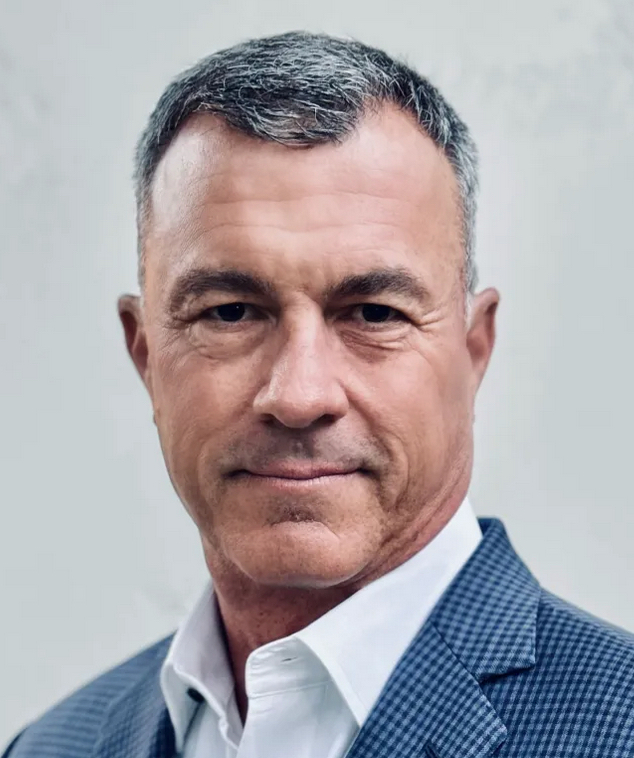
Object storage supplier Cloudian has appointed Mike Canavan as worldwide VP of sales to drive revenue growth, customer engagement, and lead all field operations across the worldwide sales team. He has a broad storage industry background, having most recently headed Americas sales at Model9, the mainframe VTL and data migration business bought by BMC. Prior to that he served as Global VP of Sales for the Emerging Solutions Business at Hitachi Vantara, and previously leading global sales for Pure Storage’s FlashBlade business. There was a stint at EMC in his CV as well. Cloudian recently took in $23 million in funding. Combine that with this appointment and it indicates Cloudian has business expansion in mind.
…
Dell and Nvidia have integrated Dell’s AI Factory with Nvidia’s Llama Stack with agentic (GenAI software that makes decisions on your behalf) workflows in mind. The reference architecture uses Dell’s PowerEdge 9680 server fitted with Nvidia H100 GPUs. Llama 3.2 introduces a versatile suite of multilingual models, ranging from 1B to 90B parameters, capable of processing both text and images. These models include lightweight text-only options (1B and 3B) as well as vision LLMs (11B and 90B), supporting long context lengths and optimized for inference with advanced query attention mechanisms. Of the updates for Meta Llama 3.2, a particularly interesting update allows enterprises to use new multimodal models to securely utilize different models for various applications, such as detecting manufacturing defects, enhancing healthcare diagnostic accuracy, and improving retail inventory management. Read about this in a Dell blog.
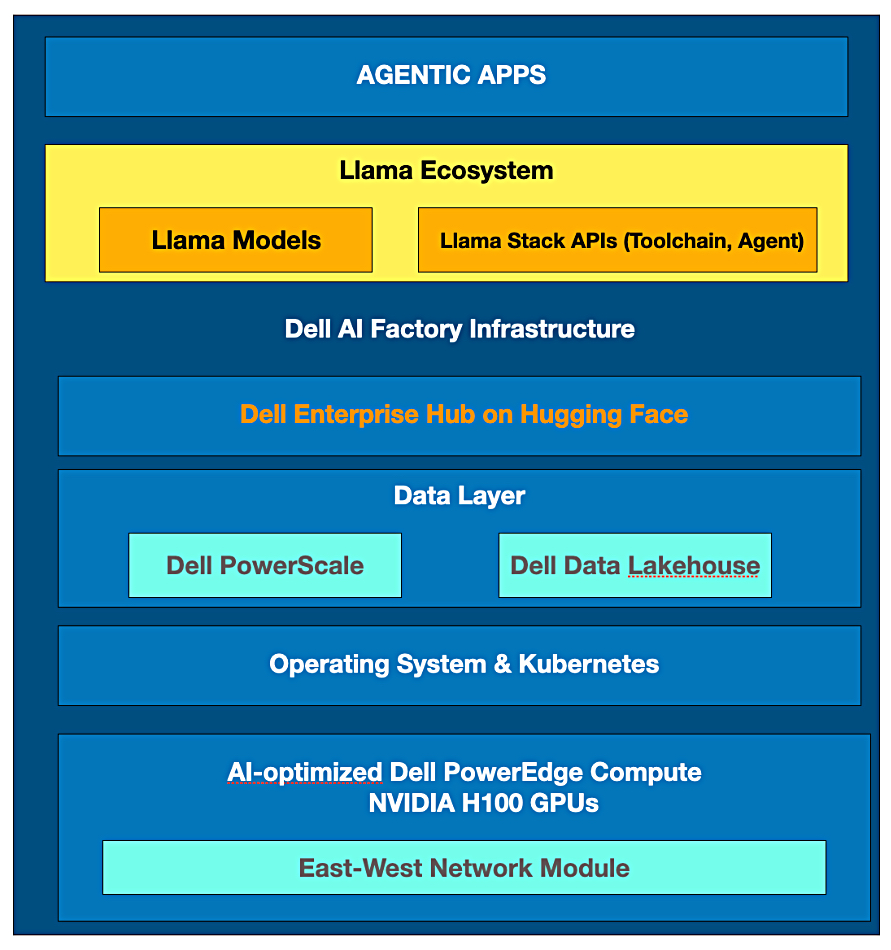
…
Data mover Fivetran has surpassed $300 million in annual recurring revenue (ARR), up from $200 million in 2023. Fivetran has consistently driven elevated ARR growth and recently reaccelerated year-over-year gains over the past two consecutive quarters.
…
HighPoint Technologies announced PCIe Gen5 and Gen4 x16 NVMe Switch series AICs and Adapters using Broadcom’s PCIe Switch ICs, supporting up to 32x 2.5-inch NVMe devices and speeds up to 60GB/sec and 7.5 million IOPS. They support features like the 2×2 drive mode for enhanced workload distribution, include Broadcom’s Hardware Secure Boot technology, and are natively supported by all modern Linux and Windows platforms. Rocket 1600 Series PCIe Gen5 x16 are equipped with Broadcom’s PEX89048 switch IC and Rocket 15xx series PCIe Gen4 x16 AICs and Adapters utilize Broadcom’s PEX88048 switch IC. Both series provide 48 lanes of internal bandwidth, and enable each AIC or Adapter to allocate 16 lanes of dedicated upstream bandwidth (to the host platform), and 4 lanes to each device port to ensure each hosted device performs optimally. The 2×2 mode splits the connection of a single NVMe SSD into two separate logical “paths,” each of which is assigned 2 PCIe lanes. The operating system will recognize each path as a distinct drive.
…
Hitachi Vantara announced significant growth in its data storage business in the first quarter of its 2024 fiscal year (ended June 30) with a 27 percent increase of quarter-over-quarter (Q/Q) product revenue growth compared to Q1 FY23, exceeding the market CAGR of 11.31 percent. The growth was even more pronounced in the United States, which saw an increase of 54 percent Q/Q compared to the previous year.
…
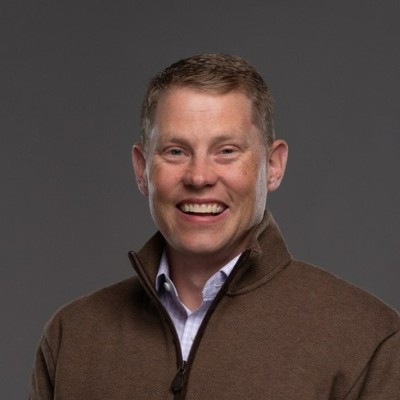
SaaS-based dat protector HYCU announced its first chief customer officer, Brian Babineau. He joins from Barracuda where he led the success and support organizations for the MSP business before becoming chief customer officer. As HYCU continues to grow its customer base from 4,200+ customers in 78 countries worldwide, Brian’s experience will be instrumental in supporting customers throughout their experience with HYCU. He was an integral part of Barracuda’s shift from an appliance to SaaS business model across several security solutions, and also has extensive experience with working with teams at scale.
…
Cloud provider Lyrid announced its open source database as a service offer based on Percona Everest. Percona announced Everest at the Open Source Summit event last week, and Lyrid is using the Everest cloud data platform to power its service. It uses Percona Everest to provide a flexible and open DBaaS where customers can choose their database and approach without the fear of vendor lock-in. This contrasts with the vast majority of DBaaS options that are tied to specific cloud providers or database options, limiting customer choice. Lyrid offers this service based on its datacenter partners, Biznet Gio and American Cloud, to provide customers with hassle-free database automation at lower costs. Customers can also choose to run this on their own datacenter environments, enjoying a fully configured and privately managed DBaaS without lock-in.
We’re told that what makes Everest unique is that it is fully open source, so any organization can run their choice of database (PostgreSQL, MySQL or MongoDB) on their preferred cloud service, including OpenStack, and on any flavor of Kubernetes as well. Using Kubernetes, Lyrid can deliver the same kind of automated database service that other DBaaS products offer, but at both lower cost and without lock-in.
…
Cloud file services suppluer Nasuni is further integrating with Microsoft 365 Copilot. Through the Microsoft Graph Connector, Nasuni managed data is fully accessible and operational with Microsoft Search and Microsoft 365 Copilot, expanding data access for Microsoft’s AI services. The Graph Connector enables organizations to leverage Nasuni’s managed data repositories, enabling Nasuni managed files to be indexed into Microsoft’s semantic index, and so provide contextually relevant answers and insights across Microsoft 365 applications. There is single-pane-of-glass access to customers’ Microsoft 365 data (including SharePoint and OneDrive) and Nasuni. This unified view allows for efficient searching and interaction with documents across the entire unstructured file stack, inclusive of Nasuni-managed data.
…
NetApp has expanded its AWS relationship with a new Strategic Collaboration Agreement (SCA) to accelerate generative AI efforts, delivering data-rich experiences through workload migration and new application deployments on AWS. The two will enable increased AWS Marketplace purchases, especially for NetApp CloudOps solutions, to streamline processes for customers. Instaclustr by NetApp manages open source vector databases – a crucial component in the delivery of fast and accurate results in RAG architectures. The close collaboration between AWS and NetApp on advanced workloads makes it simpler and faster for customers to unlock value from their data using RAG. NetApp is the only enterprise storage vendor with a first-party data storage service natively built on AWS with Amazon FSx for NetApp ONTAP.
…
Database-as-a-service (DBaaS) supplier Tessell is partnering Microsoft Azure and NetApp to deliver a ubiquitous Copilot for Cloud Databases. It integrates an enterprise-grade Database PaaS with one-click functionality for any database on Azure, leveraging Azure NetApp Files (ANF) as enterprise cloud storage and Tessell as the unified Database Service. For the first time, customers of Azure and NetApp will have access to an enterprise-grade Managed Instance for Oracle on Azure, fully integrated with ANF and supporting any virtual machine (VM) family across all Azure regions. Azure Saving Plans and NetApp effective capacity pricing are available, ensuring Co-Sell incentives and Microsoft Azure Consumption Credits (MACC) enablement. The bundled offering includes 24x7x365 support with a 15-minute response time for issues related to Azure, ANF, and Tessell Oracle PaaS.
Tessell claims customers can experience up to a 45 percent reduction in total cost of ownership (TCO) across four vectors: infrastructure optimization, third-party software optimization, database license optimization, and operational cost optimization. More information here.
…
Nutanix Unified Storage (distributed file system with commodity server-based scale-out) ranked first in the “image classification” workload category and second for the “image segmentation” workload offering top performance, faster throughput, and linear scaling in the latest round of MLPerf benchmarks, the AI storage benchmark by MLCommons. For the image classification workload, a single node was able to power 33 AI accelerators and deliver over 5,970 MB/sec throughput. The same results were achieved at scale, showing linear performance with 32-node deployment driving 1,056 AI accelerators and over 19,1043 MB/sec of throughput. Nutanix said AI is rapidly increasing the demand for sustained high-throughput and high-performance storage solutions due to the massive datasets and complex computations required for training and inference.
…
Oak Ridge National Laboratory (ORNL) is disposing of 32,494 disk drives as it is closing down its Summit supercomputer. The 20 tons of drives are being fed into a mobile ShredPro Secure shredder – a waist-high, 4 foot wide unit. The drives are fed by a technician into an opening at the top of the machine, where counter-rotating metal teeth tear the drives apart and reduce them to small, irregular strips a few inches in size. The mobile shredder can shred one hard drive every 10 seconds, with a theoretical capacity to process up to 3,500 hard drives a day. A conveyor belt gathers the material and deposits the waste into a bin, which is then transferred to larger containers and taken to be recycled through ORNL’s metal recycling program.
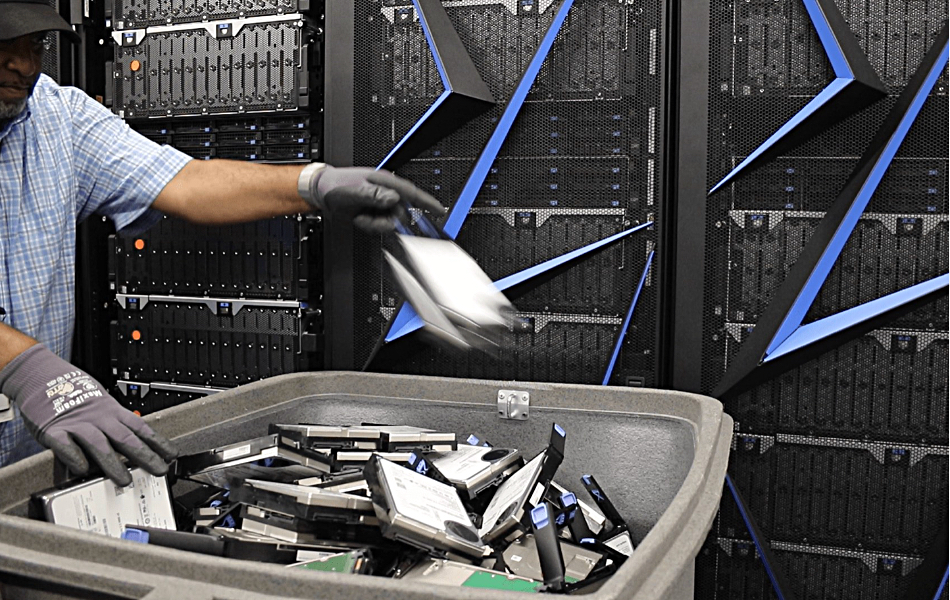
…
Pure Storage and Rubrik are partnering to add 3-layer protection to Flash Array data with a reference architecture. Layer 1 is Pure’s immutable snapshot technology, with auto-on safe mode governance providing instant recovery from a secure enclave accessible only to designated contacts authenticated through Pure Storage Support. Layer 2 is compliant immutable and on-site backup via the Rubrik Secure Vault, providing anomaly detection, threat monitoring and hunting, sensitive data monitoring, user intelligence and orchestrated recovery. Layer 3 is archival FlashBlade//S and //E storage, with massive scale-out and immutable, cost-effective storage designed for rapid recovery, even for data stored over extended periods. More info available here.
…
Rubrik has partnered with Okta to provide Okta Identity Threat Protection with user context to accelerate threat detection and response. Rubrik shares with Okta important user context such as email and the types of sensitive files they have accessed. By combining Rubrik’s Security Cloud user access risk signals with threat context from other security products used by an organization (such as Endpoint Detection and Response or EDR), Okta can determine overall risk levels more effectively and automate threat response actions to mitigate identity-based threats. A Rubrik blog provides more information.
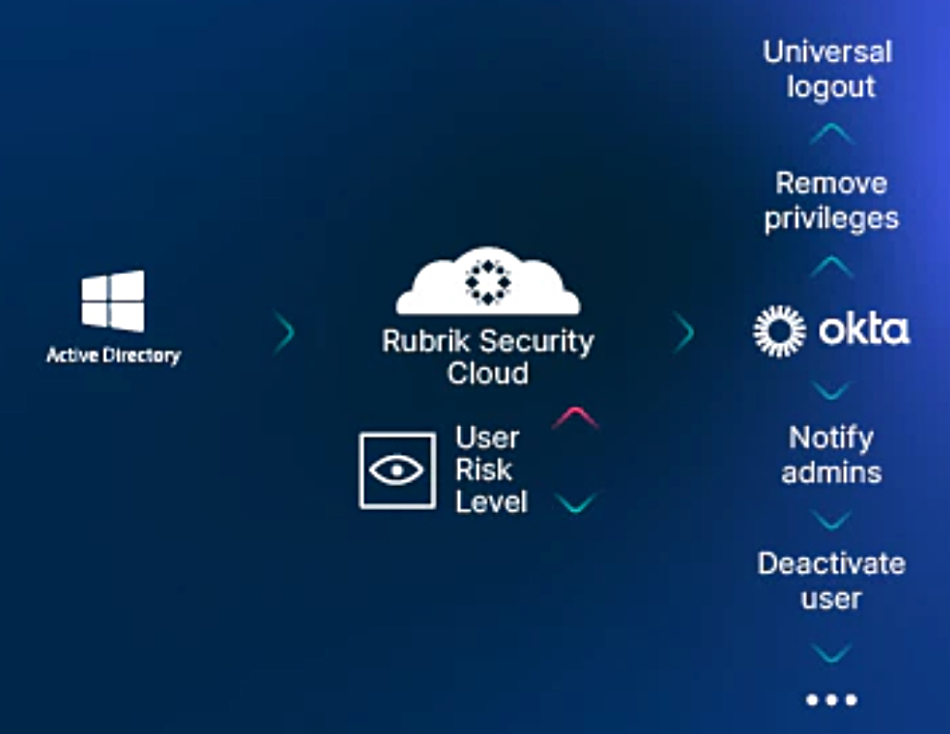
…
According to Tom’s Hardware, Samsung has announced and is mass-producing PM9E1 Gen 5 M.2 SSDs with speeds up to 14.5GB/sec read and 13GB/sec write bandwidth. The PM9E1 has a 2,400 TBW (Terabytes written) lifespan rating, double that of its PCIe 4 PM9A1 predecessor. It is also 50 percent more power-efficient. Device Authentication and Firmware Tampering Attestation security features are included through the v1.2 Security Protocol and Data Model (SPDM).
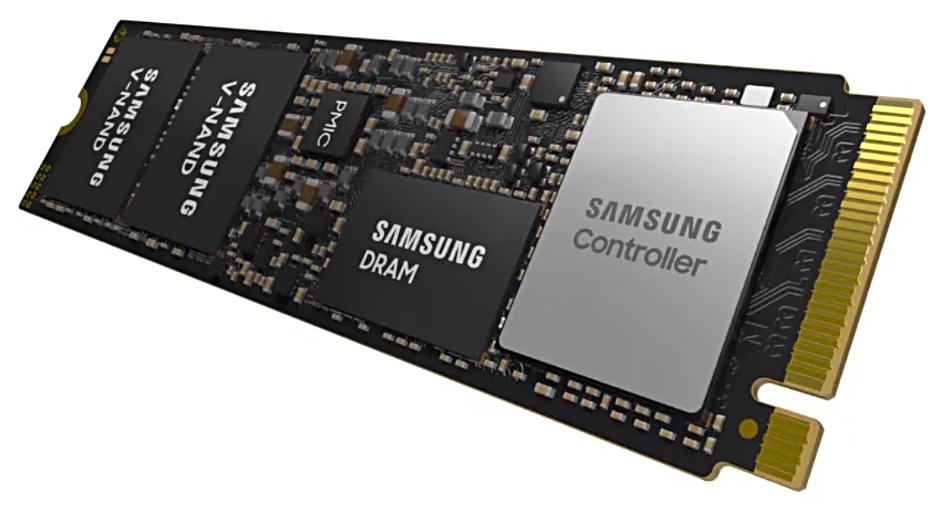
…
SK hynix has begun mass production of the world’s first 12-layer HBM3E product with the largest (36GB) capacity of existing HBM to date, using DRAM chips made 40 percent thinner to increase capacity by 50 percent at the same thickness as the previous 8-layer product. It’s increased the speed of memory operations to 9.6Gbit/sec, the highest memory speed available today. If Llama 3 70B, a Large Language Model (LLM), is driven by a single GPU equipped with four HBM3E products, it can read 70 billion total parameters 35 times within a second. Samsung Electronics and SK hynix are expected to post record sales in the third 2024 quarter, driven by AI chip demand. SK hynix could even overtake Intel in sales, becoming the third largest semiconductor supplier.
…
Enterprise app data manager Syniti is working with tyre supplier Bridgestone’s EMEA unit to remove siloes across the organization by consolidating business processes across its SAP ERP Central Component (SAP ECC) system into one SAP S/4HANA Cloud instance allowing the company to improve operational efficiency, data accuracy and set the stage for future growth. Read the full case study here.
…
Research house TrendForce claims concerns over a potential HBM oversupply in 2025 have been growing in the market. Its latest findings indicate that Samsung, SK hynix, and Micron have all submitted their first HBM3e 12-Hi samples in the first half and third quarter of 2024, respectively, and are currently undergoing validation. SK hynix and Micron are making faster progress and are expected to complete validation by the end of this year. The market is concerned that aggressive capacity expansion by some DRAM suppliers could lead to oversupply and price declines in 2025. If an oversupply does occur, it is more likely to affect older-generation products such as HBM2e and HBM3. TrendForce maintains its outlook for the DRAM industry, forecasting that HBM will account for 10 percent of total DRAM bit output in 2025, doubling its share in 2024. HBM’s contribution to total DRAM market revenue is expected to exceed 30 percent given its high ASP.
…
University of Southampton boffins, who are developing 5-dimensional silicon glass storage that basically lasts forever – it’s stable at room temperature for 300 quintillion years – stored the full human genome on a crystal of the glass which is in the Memory of Mankind archive located within a salt cave in Hallstatt, Austria. It’s the usual kind of eye-catching but useless demo of a technology that is years away from commercial use.
The boffins, led by Professor Peter Kazansky, used ultra-fast lasers to inscribe data into 20nm size nano-structured voids orientated within the silica. The crystal is inscribed with a visual key showing the universal elements (hydrogen, oxygen, carbon and nitrogen); the four bases of the DNA molecule (adenine, cytosine, guanine and thymine) with their molecular structure; their placement in the double helix structure of DNA; and how genes position into a chromosome, which can then be inserted into a cell.
The boffins’ release proclaims: “Although it is not currently possible to synthetically create humans using genetic information alone, the longevity of the 5D crystal means the information will be available if these advances are ever made in the future.” Gee whiz.
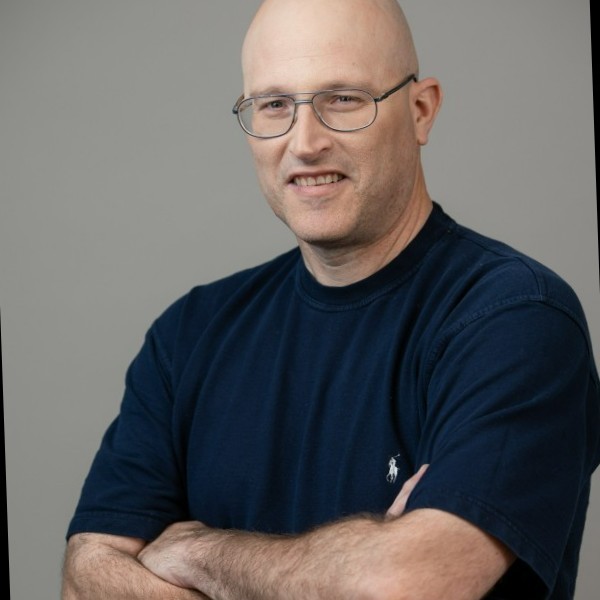
…
Open source-based hyperconverged cloud technology supplier Virtuozzo has appointed Itay Nebenzahl as its new CFO. He joins Virtuozzo from Logz.io, where he served as CFO and was instrumental in managing the company’s multi-currency cash exposure and global customer portfolio. Prior to Logz.io, Itay held the CFO position at Au10tix Limited where he led a multi-hundred-million dollar round with a leading VC, preparing the team for a multi-million special purpose acquisition company (SPAC) IPO.
…
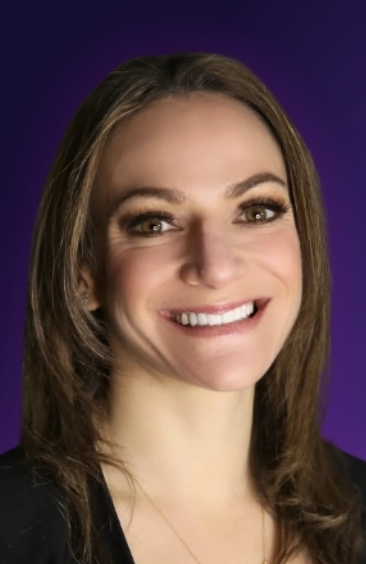
WekaIO announced that its Data Platform has been certified as a high-performance data store for Nvidia Partner Network Cloud Partners. Nvidia Cloud Partners can leverage the WEKA Data Platform’s performance, scalability, operational efficiency, and ease of use through the jointly validated WEKA Reference Architecture for Nvidia Cloud Partners using Nvidia HGX H100 systems. Also Lauren Vaccarello has been apointed as WEKA’s first CMO. Vaccarello is a veteran marketing executive and celebrated author, board member, and angel investor with a proven track record of accelerating revenue growth for enterprise software companies. She has previously served as the CMO of Salesloft and Talend and held executive leadership positions at Box, Salesforce, and Adroll. Before joining WEKA, she was an entrepreneur-in-residence at Scale Venture Partners. She sits on the boards of Thryv and USA for UNFPA.
…
Xinnor’s xiRAID technology, combined with Lustre, has enhanced HPC capabilities at German research university Friedrich-Alexander-Universität Erlangen-Nürnberg (FAU). Highlights:
- Write throughput increased from 11.3GB/sec to 67GB/sec
- Read throughput improved from 23.4GB/sec to 90.6GB/sec
- Nearly doubled usable storage capacity