DDN has demonstrated its EXAScaler storage controller software running on Nvidia’s BlueField-2 data processing units (DPUs) which replace the existing EXAScaler controllers and add a security layer to enhance data integrity.
EXAScaler is a Lustre parallel file system array. BlueField-2 and Bluefield-3 devices are host server offload accelerator systems looking after data centre networking, storage and cybersecurity capabilities.
DDN Marketing VP Kurt Kuckein wrote in a blog post yesterday: “EXAScaler Software Defined on BlueField eliminates the need for dedicated storage controllers and allows storage to scale on-demand and simply in proportion to the network.” Effectively DDN is supplying JBOD hardware and its EXAScaler software which runs in the Arm-powered BlueField-2 SoC.
This EXAScaler-BlueField integration was demonstrated at Nvidia’s virtual GPU Technology Conference on April 12.
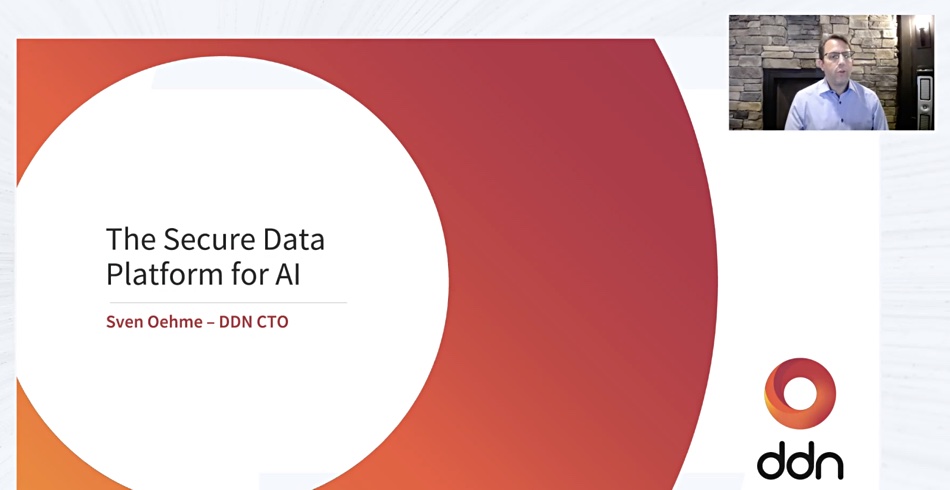
Sven Oehme, DDN CTO, presented a session titled ‘The Secure Data Platform for AI’, during which he demonstrated an EXAScaler/BlueField-2 array linked to an Nvidia DGX GPU server and feeding it with data.
Oehme said that running the EXAScaler storage stack on BlueField meant less network management complexity, with BlueField managing everything above the base physical connectivity layer. BlueField also provided security measures that isolated the EXAScaler storage and its data from attackers.
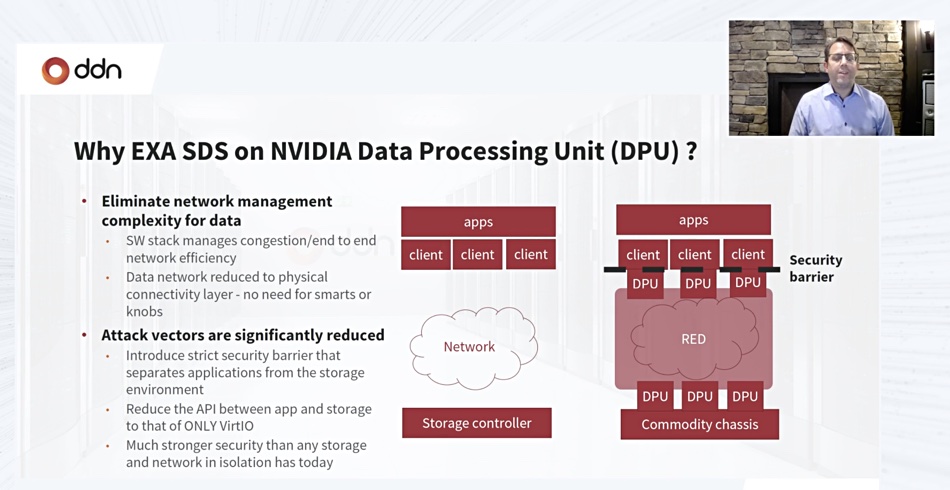
Kuckein wrote: “IO data now inherits all the benefits of BlueField’s network isolation, significantly reducing the number of attack vectors available to a malicious actor. Right away, hacked user accounts, rogue users, man in the middle attacks, hacked root accounts and other avenues for malicious activity are eliminated.” [His emphasis.]
The EXAScaler software stack was split in the demo between array (server) software running in the array’s BlueField-2 DPU and client (DGX) software running in the DGX GPU server’s BlueField-2 DPU. There was effectively a private 200Gbit/s network link between the two BlueField cards.
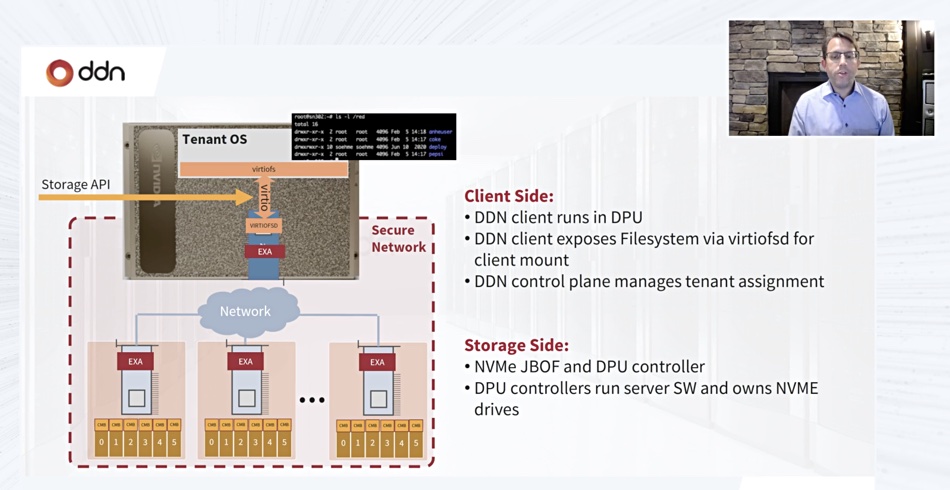
The demo showed multiple tenants, with each tenant seeing only their portion of the EXAScaler datastore and making virtual API calls to the EXAScaler array.
Oehme said the initial software port to Bluefield-2 has been completed. Over the next couple of quarters, more DDN EXAScaler software releases will provide integration with server CPU bypass GPUDirect storage, end-to-end zero copy, and application Quality of Service.
The end-to-end zero copy means that data is transmitted from and to the array and the DGX with no time-consuming intermediate copying for data preparation at either end.
Comment
Here we have DDN’s EXAScaler array software running on proprietary Nvidia hardware. We don’t know how the performance of the BlueField-2 hardware (8 x Arm A72 cores) compares to the existing EXAScaler dual active:active Xeon controllers but suspect it’s a lot less.
An EXAScaler array using DDN’s SFA18KX hardware has four gen 2 Xeon Scalable Processors and delivers up to 3.2 million IOPS and transfers data at 90GB/sec.
We suspect that Bluefield-3, with 16 x Arm A78 cores, would be needed to approach this performance level.
When DDN and Nvidia add GPUDirect support to EXAScaler BlueField, that could provide DDN with a lower cost product than other storage suppliers supporting GPUDirect, such as Pavilion Data, WekaIO and VAST Data. The EXAScaler-BlueField-GPUDirect performance numbers will be very interesting to see.
Another angle to this DDN EXAScaler BlueField port lies in asking the question; which other storage suppliers could do likewise? DDN has said both NetApp and WekaIO are BlueField ecosystem members. Will either port their software to BlueField?
Lastly, Blocks & Files sees a parallel between DDN porting its EXAScaler storage software to a directly-connected and Arm-powered DPU and Nebulon developing its own Arm-powered Storage Processing Unit (SPU) hardware to provide storage array functionality using SSDs in the server hosting the Nebulon SPU card. The storage software stack runs in the DPU/SPU card and reads and writes data to drives in an attached chassis – JBOF with DDN, and server with Nebulon.