Commvault is announcing new cloud support and anti-malware updates at its London Shift customer event, with full Commvault Cloud availability on AWS, protection of the Google Workspace suite, point-in-time recovery and rebuild of SaaS suite backups, as well as a partnership with Pure Storage to support financial customers affected by the EU’s Digital Operational Resilience Act (DORA).
Commvault Cloud is the new branding for its Metallic SaaS data protection service. DORA is a set of EU regulations to enhance the cyber resilience of financial institutions aiming to ensure they can continue to function during cyberattacks or other potentially disastrous IT incidents. It is scheduled to come into force from January 2025.
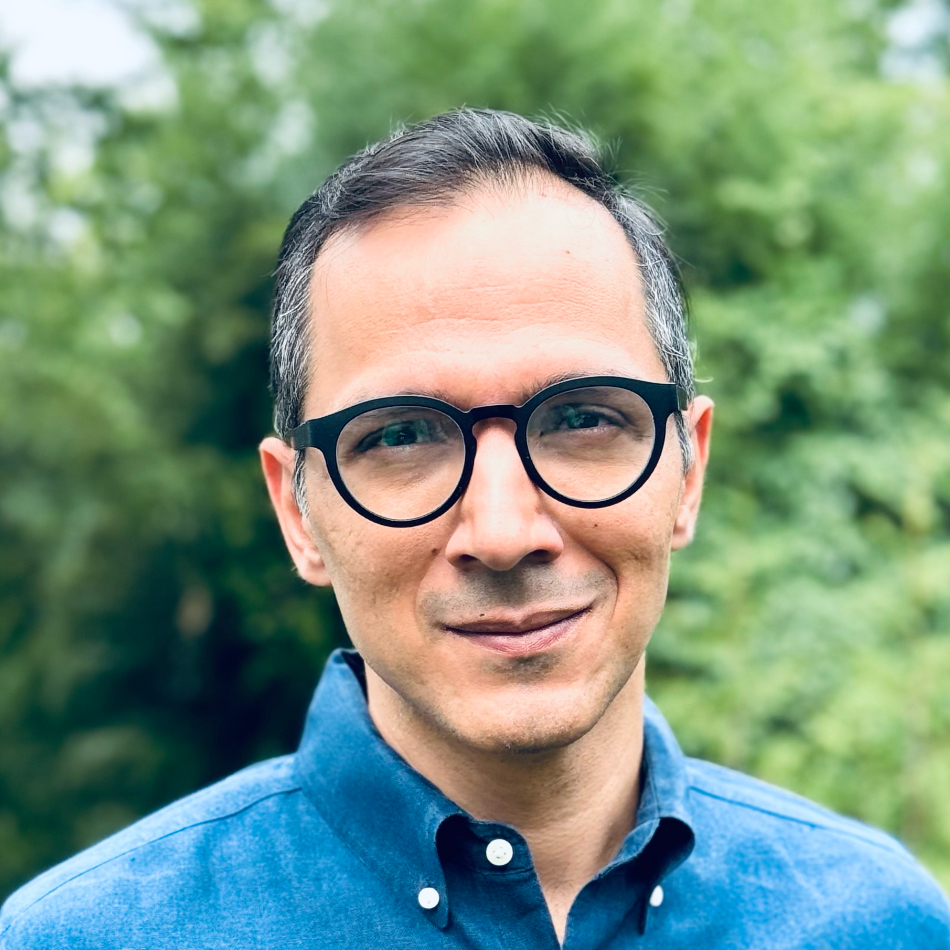
Pranay Ahlawat, Commvault Chief Technology and AI Officer, stated: “We are proud to extend the full power of the Commvault Cloud platform and cloud-native solutions to AWS. We believe our game-changing technology will empower joint customers to recover faster, mitigate threats more effectively, and enhance their cyber resilience strategies.”
Offerings that will be available to AWS customers include:
- Cloud Rewind: Based on Appranix technology, Cloud Rewind acts as an AWS time machine, enabling customers to “rewind” to the last clean copy of their data, recover that data, and automate the cloud application rebuild process so that businesses can get back to normal in minutes.
- Cyber Resilience for Amazon S3: Through its recent acquisition of Clumio, Commvault will be introducing new technology in the coming months that will also bring time machine capabilities to Amazon Simple Storage Service (S3) customers. In the event of an attack, this technology will allow customers to revert quickly to to a clean copy of data that has not been infiltrated with malware.
- Air Gap Protect: This will provide AWS customers with immutable, isolated copies of data in a Commvault tenant as a service, giving AWS customers another way to keep their data safe and resilient.
- Cleanroom Recovery: This is already present in Azure and being extended to AWS. It will allow organizations to automatically provision recovery infrastructure, enabling recovery to an isolated location in AWS and restore infrastructure workloads like Active Directory and production workloads. They can also conduct forensics in this clean, safe location, and it enables IT and security teams to test their cyber recovery plans in advance so that they know that when they are hit, they can recover quickly.
Cloud Rewind is unique to Commvault. “When organizations are attacked, restoring the data is only half the battle,” the company says. “The truly laborious task is actually restoring the distributed cloud applications, which are used to run and power that data.”
A typical enterprise could run more than 350 SaaS apps and attack recovery can require these apps to be restored in a systematic way that takes a lot of time when done manually. Appranix technology automatically identifies and catalogs all cloud components in use, offering full visibility into what assets need protection and recovery, analyzing and defining the relationships and dependencies between various cloud components.
It builds an operational blueprint, capturing the data and the full map of applications, infrastructure, and networking configurations so that “systems can be restored with their complete operational blueprint intact, reducing guesswork for a thorough recovery … When a system is restored, all connected resources and services are aligned, with little to no human involvement.”
Cloud Rewind supports all major public and private cloud platforms, including AWS, Google Cloud, and Microsoft Azure.
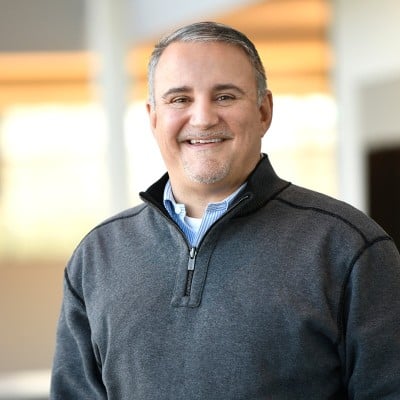
Brian Brockway, Commvault CTO, stated: “What we are doing with Cloud Rewind is unlike anything offered on the market today. In the ransomware era, recovering data is important, but it’s table stakes. We’re ushering in an entirely new chapter in cyber resilience that not only expedites data recovery, but recovery of cloud applications. This is the gold standard in recovery for a cloud-first world.”
A new Cyber Resilience Dashboard provides continuous ransomware readiness assessments and indicates gaps in resilience plans. It provides a view across the entire data estate, assessing components such as testing frequency and success, and availability of immutable air-gapped copies of critical data.
We understand that a coming Clumio capability will be the instant restore of massive S3 buckets with tens of billions of objects. Restoring single S3 objects has easy but restoring buckets at massive scale is a different and vastly more difficult proposition. Clumio’s tech uses snapshotting and versioning with the ability to go back to a point in time and restore a bucket’s state.
Google Cloud
Commvault has launched SaaS-based Cloud Backup & Recovery for Google Workspace. This provides Gmail, Google Drive, Shared Drives, and built-in Google Cloud Storage for Google Workspace protection. Google Workspace customers will be able to effortlessly discover active data, rapidly recover from inadvertent or malicious data deletion, and maintain a copy of valuable data in the Commvault Cloud for compliance mandates.
It is expanding its Google Cloud capabilities with Cloud Rewind, which integrates Appranix’s application rebuild capabilities into the Commvault Cloud platform, providing Google Cloud customers with an automated, cloud-native rebuild solution to recover from cyber incidents more rapidly.
Earlier this year, Commvault announced support for object retention lock for Google Cloud Storage, providing customers with immutable cloud storage on Google Cloud infrastructure.
Pure Storage
Commvault announced a joint cyber-readiness offering with Pure Storage to deliver the ability to continuously test recovery in secure, isolated environments; on-demand in cloud-isolated tenants via Commvault’s Cleanroom Recovery solution or within isolated recovery environments. The two say that customers “can easily deliver rapid, frictionless recovery of clean data to isolated environments with the flexibility needed to meet operational and data sovereignty requirements.”
This is not only applicable to DORA, but supports compliance under other cybersecurity and privacy regulations like the EU’s NIS2 Directive and e-mandates from Reserve Bank of India (RBI) for recurring transactions.
Patrick Smith, Field CTO, EMEA, Pure Storage, said: “Through our partnership with Commvault, we are giving financial institutions critical tools that not only help comply with regulations like DORA but advance their cyber resilience to help ensure enterprise data remains secure, protected, and if necessary, recoverable.”
Availability
Commvault’s suite of offerings for AWS will be generally available in the coming months. Following that, joint customers will be able to access them in the AWS Marketplace. Commvault Cloud Backup & Recovery for Google Workspace is targeted for availability by the end of the calendar year. Cloud Rewind and the Cyber Resilience Dashboard will be generally available in the coming months.