Strong sequential growth in storage revenues at Dell was not enough to prevent an 11 percent revenue fall for the final fiscal 2024 quarter as the company waits in hope of an AI-driven recovery.
Dell reported $22.3 billion in revenues for the quarter ended February 2, with a $1.2 billion profit, up 91 percent year-on-year. Full year revenues were $88.4 billion, down 13.5 percent from the year before, with a profit of $3.2 billion, 32 percent higher year-on-year. Squeezing more profit from lower revenues is quite the achievement.
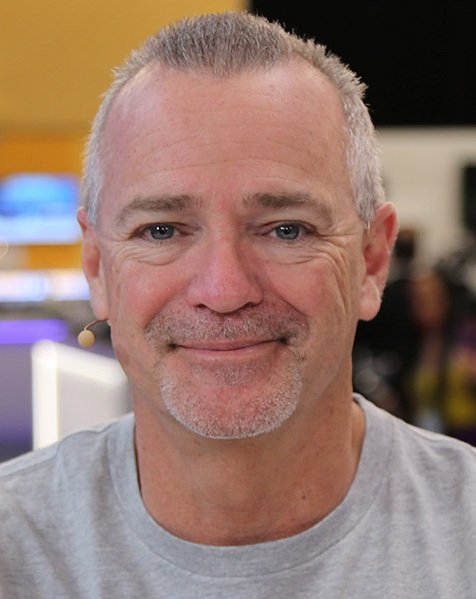
Vice chairman and COO Jeff Clarke said in prepared remarks: “In a year where revenue declined, we maintained our focus on operational excellence delivering solid earnings per share and outstanding cash flow. FY24 was one of those years that didn’t go as planned, but I really like how we navigated it. We showed our grit and determination by quickly adapting to a dynamic market, focusing on what we can control, and extending our model into the high growth AI opportunity.”
CFO Yvonne McGill said: “We generated $8.7 billion in cash flow from operations this fiscal year, returning $7 billion to shareholders since Q1 FY23. We’re optimistic about FY25 and are increasing our annual dividend by 20 percent – a testament to our confidence in the business and ability to generate strong cash flow.”
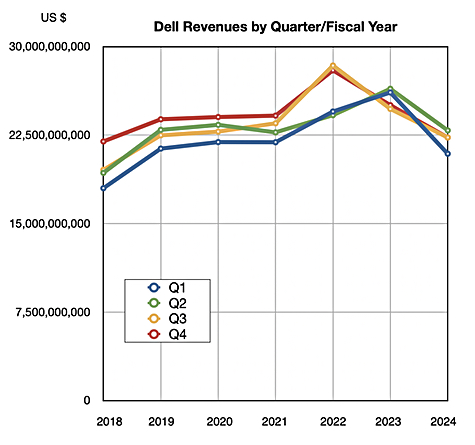
Quarterly financial summary
- Gross margin: 23.8 percent vs 23 percent a year ago
- Operating cash flow: $1.5 billion
- Free cash flow: $1 billion vs $2.3 billion last year; 55 percent lower
- Cash, cash equivalents, and restricted cash: $7.5 billion vs $8.9 billion last year
- Diluted earnings per share: $1.59
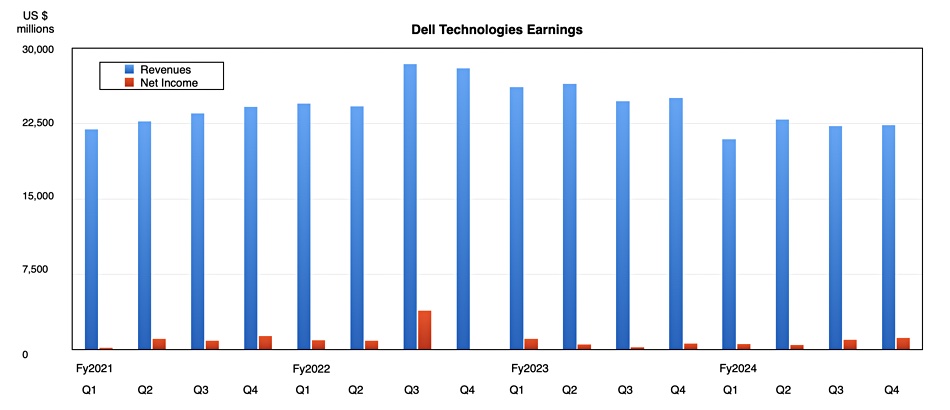
Dell has two main business units – Infrastructure Solutions Group (ISG) and Client Solutions Group (CSG). The larger CSG, with its PCs and laptops, had revenues of $11.7 billion, 12 percent down year-on-year, while ISG, with its servers, storage and networking, reported $9.3 billion, six percent lower annually.
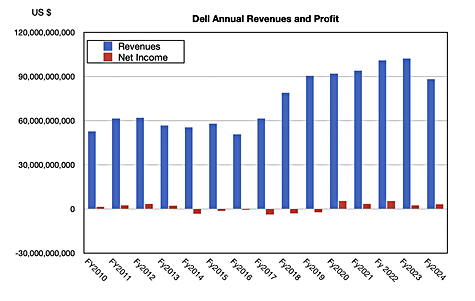
Servers and networking brought in $4.9 billion, the same as a year ago, while storage was responsible for $4.5 billion, down 10 percent year-on-year but up 16 percent quarter-on-quarter.
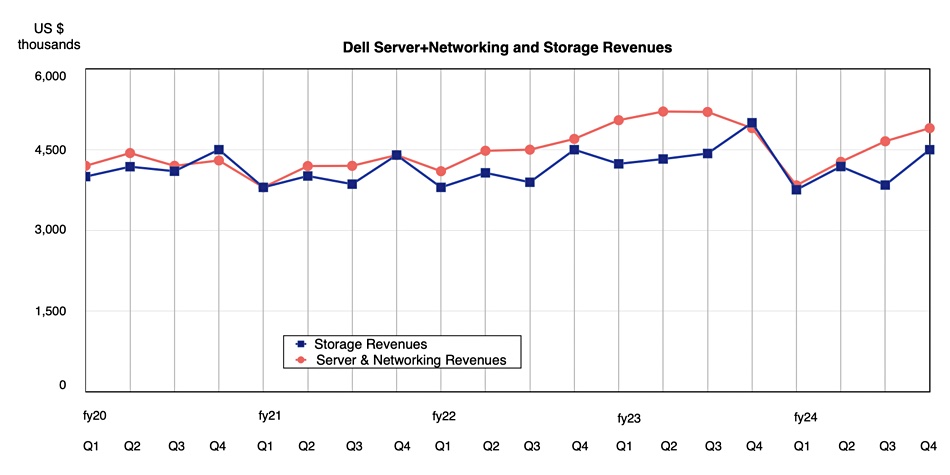
Clarke said: “Our strong AI-optimized server momentum continues, with orders increasing nearly 40 percent sequentially and backlog nearly doubling, exiting our fiscal year at $2.9 billion,” implying that the next few quarter’s results should be better.
“We’ve just started to touch the AI opportunities ahead of us, and we believe Dell is uniquely positioned with our broad portfolio to help customers build GenAI solutions that meet performance, cost and security requirements.”
Why the quarterly storage revenue rise? Dell said there was demand strength across the portfolio, more than any expected seasonal improvement. Clarke said on the earnings call: “We had year-over-year demand growth in the unstructured space, ECS, as well as PowerScale. They grew quarter-over-quarter and year-over-year on a demand basis. Those are generally good indicators … around AI file and object, which are the data classes that generally feed the AI engines … Our progress in traditional storage was good too. We were ahead of our normal seasonality … It was down year-over-year, but better than we expected across mid-range, across our data protection products and our high-end storage products.”
The outlook is for growth. Clarke said: “We believe the long-term AI action is on-prem where customers can keep their data and intellectual property safe and secure. PCs will become even more essential as most day-to-day work with AI will be done on the PC. We remain excited about the long-term opportunity in our CSG business.”
He added: “Our storage business will benefit from the exponential growth expected in unstructured data … We think AI moves to the data. More data will be created outside of the data center going forward than inside the data center today. That’s going to happen at the edge of the network. A smart factory, an oil derrick or platform, a deep mine, all variations of this. We believe AI will ultimately get deployed next to where the data is created driven by latency.”
In his view, enterprises will “quickly find that they want to run AI on-prem because they want to control their data. They want to secure their data. It’s their IP and they want to run domain specific and process specific models to get the outcomes they’re looking for.”
Dell thinks an AI-fueled recovery in storage demand will lag stronger server demand by a couple of quarters.
Revenues for Q1 FY25 are expected to be between $21 billion and $22 billion, 3 percent higher annually at the midpoint. But then growth will accelerate. Clarke sees “modest growth in traditional [servers], stronger growth in AI-enabled servers, and an opportunity with storage as the year progresses.”
Full FY25 revenues should be between $91 billion and $95 billion, up 5 percent year-on-year at the midpoint. McGill said: “We expect ISG to grow in the mid-teens fueled by AI, with a return to growth in traditional servers and storage, and our CSG business to grow in the low single digits for the year.”