Amazon has re-engineered the AWS EBS stack to enable on-premises levels of SAN performance in the cloud. Make no mistake, the cloud giant is training its big guns at the traditional on-premises storage area networking vendors.
The company revealed yesterday at re:Invent 2020 that it has separated the Elastic Block Store compute and storage stacks at the hardware level so they can scale at their own pace. AWS has also rewritten the networking stack to utilise its high-performance Scalable Reliable Datagrams (SRD) networking protocol, and so lower latency.
The immediate fruits of this architecture overhaul include EBS Block Express, the “first SAN built for the cloud”. AWS said the service is “designed for the largest, most I/O intensive mission-critical deployments of Oracle, SAP HANA, Microsoft SQL Server, and SAS Analytics that benefit from high-volume IOPS, high throughput, high durability, high storage capacity, and low latency.”
Pure conjecture from us, but Amazon could hit the SAN storage suppliers squarely in their own backyards by introducing EBS Block Express to the AWS Outposts on-premise appliance.
Mai-Lan Tomsen Bukovec, VP Storage, at AWS, said in a statement: “Today’s announcements reinvent storage by building a new SAN for the cloud, automatically tiering customers’ vast troves of data so they can save money on what’s not being accessed often, and making it simple to replicate data and move it around the world as needed to enable customers to manage this new normal more effectively.”
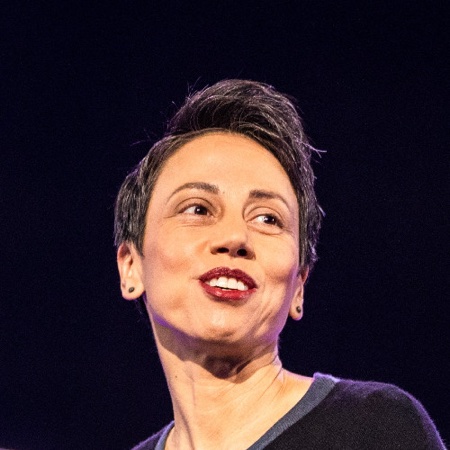
AWS noted that many customers had previously striped multiple EBS io2 volumes together to achieve higher IOPS, throughput or capacity. But this is sub-optimal. The alternative – on-premises SANS – are “expensive due to high upfront acquisition costs, require complex forecasting to ensure sufficient capacity, are complicated and hard to manage, and consume valuable data center space and networking capacity”
Now EBS io2 Block Express volumes can support up to 256,000 IOPS, 4,000 MB/second of throughput, and 64TB of capacity. This is a fourfold increase over existing io2 volumes across all parameters. The new volumes have sub-millisecond latency and users can stripe multiple io2 Block Express volumes together to get better performance.
Decoupled compute and storage
AWS yesterday said decoupling of compute and storage in the EBS service has enabled it introduce a new class of Gp (general purpose) volume for general purpose workloads such as relational and non relational databases. Capacity grows in lockstep with improvements in performance ((IOPS and throughput) with the existing Gp2 volumes and this means customers can end up paying for storage that they don’t need.
AWS has addressed this with Gp3 volumes, to enable users to utilise a claimed 4x performance increase over Gp2 volumes – without incurring a storage tax. As well as independent scaling, Gp3 volumes are priced 20 per cent cheaper than Gp2. Migration from Gp2 to Gp3 is seamless, AWS says, and handled via Elastic Volumes.
Tiering and replication
The Archive Access (S3 Glacier) and Deep Archive Access (S3 Glacier Deep Archive) tiers, announced in November with S3’s Intelligent-Tiering, are now generally available. Customers can lower storage costs by putting cold data into progressively deeper and lower-cost AWS archives.
S3 Replication enables the creation of a replica copy of customer data within the same AWS Region or across different AWS Regions. This is now extended to replicate data to multiple buckets within the same AWS Region, across multiple AWS Regions, or a combination of both.
AWS io2 Block Express volumes are available in limited preview.