Cohesity announced it is the first data protection provider to achieve Nutanix’s Database Service (NDB) database protection Nutanix Ready validation. It says: “NDB is the market leading database lifecycle management platform for building database-as-a-service solutions in hybrid multicloud environments.” Cohesity DataProtect now integrates with NDB’s native time machine capabilities and streamlines protection for PostgreSQL databases on NDB via a single control plane.
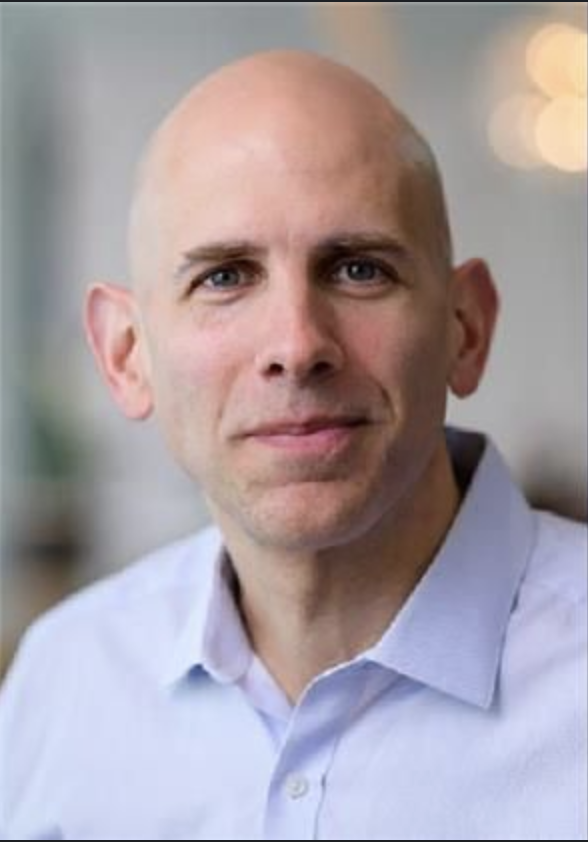
…
Data protector Commvault has announced the appointment of Bill O’Connell as its chief security officer. He had prior leadership roles at Roche, leading technical, operational, and strategic programs to protect critical data and infrastructure, and also at ADP. He previously served as chair of the National Cyber Security Alliance Board of Directors and remains actively involved in various industry working groups focused on threat intelligence and privacy.
…
Global food and beverage company Danone has adopted Databricks’ Data Intelligence Platform to drive improvements in data accuracy and reduce “data-to-decision” time by up to 30 percent. It says data ingestion times are set to drop from two weeks to one day, with fewer issues requiring debugging and fixes. A “Talk to Your Data” chatbot, powered by generative AI and Unity Catalog, will help non-technical users explore data more easily. Built-in tools will support rapid prototyping and deployment of AI models. Secure, automated data validation and cleansing could increase accuracy by up to 95 percent.
…
ExaGrid announced three new models, adding the EX20, EX81, and EX135 to its line of Tiered Backup Storage appliances, as well as the release of ExaGrid software version 7.2.0. The EX20 has 8 disks that are 8 TB each. The EX81 has 12 disks that are 18 TB each. The EX135 has 18 disks that are 18 TB each. Thirty-two of the EX189 appliances in a single scale-out system can take in up to a 6 PB full backup with 12 PB raw capacity, making it the largest single system in the industry that includes data deduplication. ExaGrid’s line of 2U appliances now include eight models: EX189, EX135, EX84, EX81, EX54, EX36, EX20, and EX10. Up to 32 appliances can be mixed and matched in a single scale-out system. Any age or size appliance can be used in a single system, eliminating planned product obsolescence.
The product line has also been updated with new Data Encryption at Rest (SEC) options. ExaGrid’s larger appliance models, including the EX54, EX81, EX84, EX135, and EX189, offer a Software Upgradeable SEC option to provide Data Encryption at Rest. SEC hardware models that provide Data Encryption at Rest are also available for ExaGrid’s entire line of appliance models. The v7.20 software includes External Key Management (EKM) for encrypted data at rest, support for NetBackup Flex Media Server Appliances with the OST plug-in, support of Veeam S3 Governance Mode and Dedicated Managed Networks.
…
Data integration provider Fivetran announced it offers more than 700 pre-built connectors for seamless integration with Microsoft Fabric and OneLake. This integration, powered by Fivetran’s Managed Data Lake Service, enables organizations to ingest data from over 700 connectors, automatically convert it into open table formats like Apache Iceberg or Delta Lake, and continuously optimize performance and governance within Microsoft Fabric and OneLake – without the need for complex engineering effort.
…
Edge website accelerator Harper announced version 5 of its global application delivery platform. It includes several new features for building, scaling, and running high-performance data-intensive workloads, including the addition of Binary Large Object (Blob) storage for the efficient handling of unstructured, media-rich data (images, real-time videos, and rendered HTML). It says the Harper platform has unified the traditional software stack – database, application, cache, and messaging functions – into a single process on a single server. By keeping data at the edge, Harper lets applications avoid the transit time of contacting a centralized database. Layers of resource-consuming logic, serialization, and network processes between each technology in the stack are removed, resulting in extremely low response times that translate into greater customer engagement, user satisfaction, and revenue growth.
…
Log data lake startup Hydrolix has closed an $80 million C-round of funding, bringing its total raised to $148 million. It has seen an eightfold sales increase in the past year, with more than 400 new customers, and is building sales momentum behind a comprehensive channel strategy. The cornerstone of that strategy is a partnership with Akamai, whose TrafficPeak offering is a white label of Hydrolix. Additionally, Hydrolix recently added Amazon Web Services as a go-to-market (GTM) partner and built connectors for massive log-data front-end ecosystems like Splunk. These and similar efforts have driven the company’s sales growth, and the Series C is intended to amplify this momentum.
…
Cloud data management supplier Informatica has appointed Krish Vitaldevara as EVP and chief product officer coming from NetApp and Microsoft. This is a big hire. He was an EVP and GM for NetApp’s core platforms and led NetApp’s 2,000-plus R&D team responsible for technology, including ONTAP, FAS/AFF, application integration & data protection software. At Informatica, “Vitaldevara will develop and execute a product strategy aligning with business objectives and leverage emerging technologies like AI to innovate and improve offerings. He will focus on customer engagement, market expansion and strategic partnerships while utilizing AI-powered, data-driven decision-making to enhance product quality and performance, all within a collaborative leadership framework.”
…
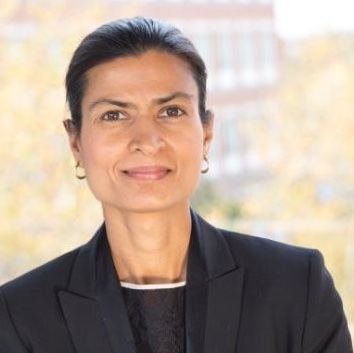
Cloud file services supplier Nasuni has appointed Sam King as CEO, succeeding Paul Flanagan who is retiring after eight years in the role. Flanagan will remain on the Board, serving as Non-Executive Chairman. King was previously CEO of application security platform supplier Veracode from 2019 to 2024.
…
ObjectFirst has announced three new Ootbi object backup storing appliances for Veeam, with new entry-level 20 and 40 TB capacities, and a range-topping 432 TB model, plus new firmware delivering 10-20 percent faster recovery speeds across all models. The 432 TB model supports ingest speeds of up to 8 GBps in a four-node cluster, double the previous speed. New units are available for purchase immediately worldwide.
…
OpenDrives is bringing a new evolution of its flagship Atlas data storage and management platform to the 2025 NAB Show. Atlas’ latest release provides cost predictability and economical scalability with an unlimited capacity pricing model, high performance and freedom from paying for unnecessary features with targeted composable feature bundles, greater flexibility and freedom of choice with new certified hardware options, and intelligent data management via the company’s next-generation Atlas Performance Engine. OpenDrives has expanded certified hardware options to include the Seagate Exos E JBOD expansion enclosures.
…
Other World Computing announced the release of OWC SoftRAID 8.5, its RAID management software for macOS and Windows, “with dozens of enhancements,” delivering “dramatic increases in reliability, functionality, and performance.” It also announced the OWC Archive Pro Ethernet network-based LTO backup and archiving system with drag-and-drop simplicity, up to 76 percent cost savings versus HDD storage, a 501 percent ROI, and full macOS compatibility.
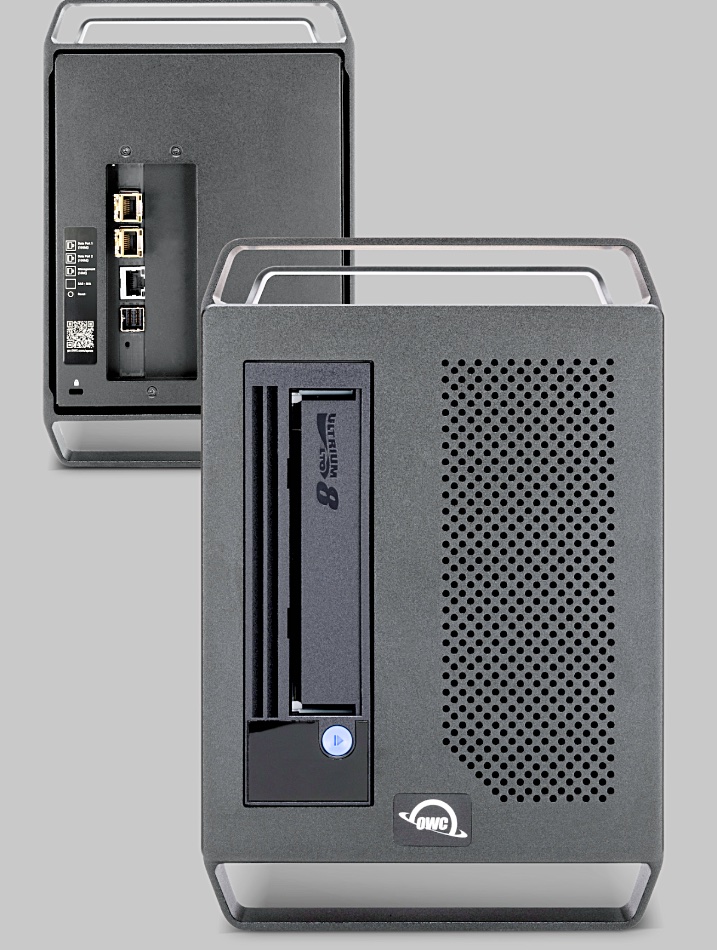
…
Percona is collaborating with Red Hat on OpenShift and Percona Everest will now support OpenShift, so you can run a fully open source platform for running “database as a service” style instances on your own private or hybrid cloud. The combination of Everest as a cloud-native database platform with Red Hat OpenShift allows users to implement their choice of database in their choice of locations – from on-premises datacenter environments through to public cloud and hybrid cloud deployments.
…
Perforce Delphix announced GA of Delphix Compliance Services, a data compliance product built in collaboration with Microsoft. It offers automated AI and analytics data compliance supporting over 170 data sources and natively integrated into Microsoft Fabric pipelines. The initial release of Delphix Compliance Services is pre-integrated with Microsoft Azure Data Factory and Microsoft PowerBI to natively protect sensitive data in Azure and Fabric sources as well as other popular analytical data stores. The next phase of this collaboration adds a Microsoft Fabric Connector.
Perforce is a Platinum sponsor at the upcoming 2025 Microsoft Fabric Conference (FabCon) jointly sponsoring with PreludeSys. It will be demonstrating Delphix Compliance Services and natively masking data for AI and analytics in Fabric pipelines at booth #211 and during conference sessions.
…
Pliops has announced a strategic collaboration with the vLLM Production Stack developed by LMCache Lab at the University of Chicago, aimed at revolutionizing large language model (LLM) inference performance. The vLLM Production Stack is an open source reference implementation of a cluster-wide full-stack vLLM serving system. Pliops has developed XDP (Extreme Data Processor) key-value store technology with its AccelKV software running in an FPGA or ASIC to accelerate low-level storage stack processing, such as RocksDB. It has announced a LightningAI unit based on this tech. The aim is to enhance LLM inference performance.
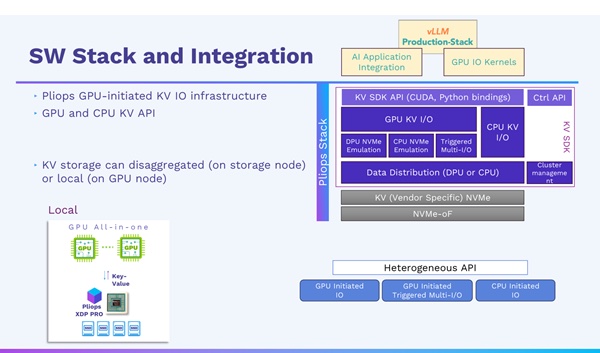
…
Pure Storage is partnering with CERN to develop DirectFlash storage for Large Hadron Collider data. Through a multi-year agreement, Pure Storage’s data platform will support CERN openlab to evaluate and measure the benefits of large scale high-density storage technologies. Both organizations will optimize exabyte-scale flash infrastructure, and the application stack for Grid Computing and HPC workloads, identifying opportunities to maximize performance in both software and hardware while optimizing energy savings across a unified data platform.
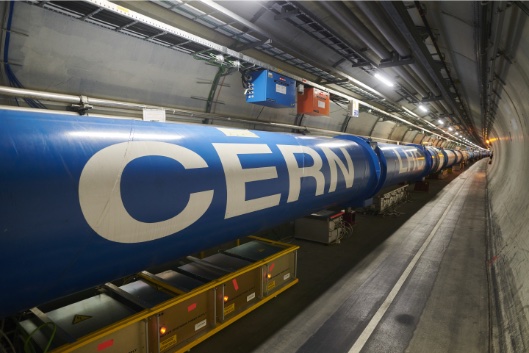
…
Seagate has completed the acquisition of Intevac, a supplier of thin-film processing systems for $4.00 per share, with 23,968,013 Intevac shares being tendered. Intevac is now a wholly owned subsidiary of Seagate. Wedbush said: “We see the result as positive for STX given: 1) we believe media process upgrades are required for HAMR and the expense of acquiring and operating IVAC is likely less than the capital cost for upgrades the next few years and 2) we see the integration of IVAC into Seagate as one more potential hurdle for competitors seeking to develop HAMR, given that without an independent IVAC, they can no longer leverage the sputtering tool maker’s work to date around HAMR (with STX we believe using IVAC exclusively for media production.”
…
DSPM provider Securiti has signed a strategic collaboration agreement (SCA) with Amazon Web Services (AWS). AWS selected Securiti to help enterprise customers safely use their data with Amazon Bedrock’s foundation models, integrating Securiti’s Gencore AI platform to enable compliant, secure AI development with structured and unstructured data. Securiti says its Data Command Graph provides contextual data intelligence and identification of toxic combinations of risk, including the ability to correlate fragmented insights across hundreds of metadata attributes such as data sensitivity, access entitlements, regulatory requirements, and business processes. It also claims to offer the following:
- Advanced automation streamlines remediation of data risks and compliance with data regulations.
- Embedded regulatory insights and automated controls enable organizations to align with emerging AI regulations and frameworks such as EU AI Act and NIST AI RMF.
- Continuous monitoring, risk assessments and automated tests streamline compliance and reporting.
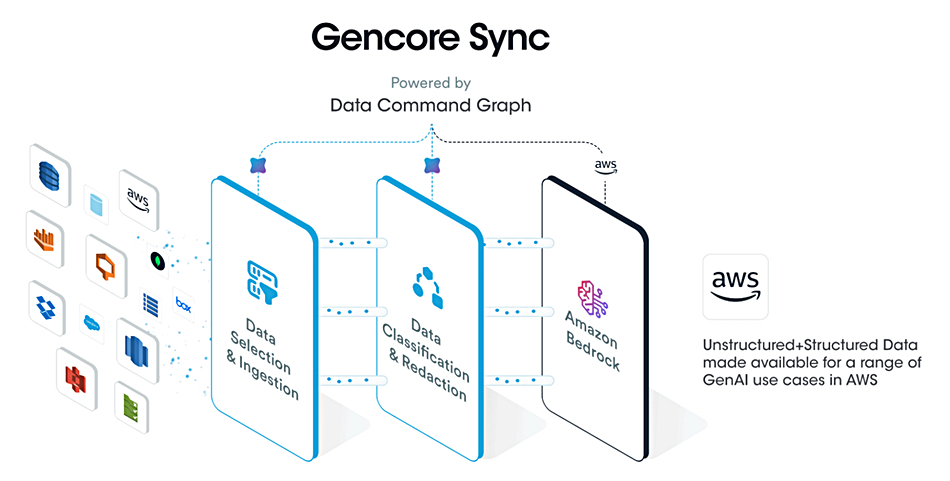
…
SpectraLogic has launched the Rio Media Suite, which it says is simple, modular and affordable software to manage, archive and retrieve media assets across a broad range of on-premises, hybrid and cloud storage systems. It helps break down legacy silos, automates and streamlines media workflows, and efficiently archives media. It is built on MediaEngine, a high-performance media archiver that orchestrates secure access and enables data mobility between ecosystem applications and storage services.
A variety of app extensions integrate with MediaEngine to streamline and simplify tasks such as creating and managing lifecycle policies, performing partial file restores, and configuring watch folders to monitor and automatically archive media assets. The modular MAP design of Rio Media Suite allows creative teams to choose an optimal set of features to manage and archive their media, with the flexibility to add capabilities as needs change or new application extensions become available.
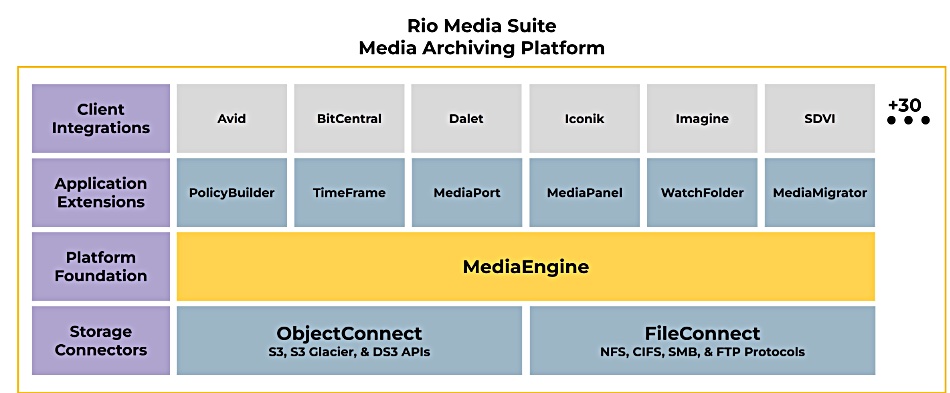
Available object and file storage connectors enable a range of Spectra Logic and third-party storage options, including Spectra BlackPearl storage systems, Spectra Object-Based Tape, major third-party file and object storage systems, and public cloud object storage services from leading providers such as AWS, Geyser Data, Google, Microsoft and Wasabi.
A live demonstration of Rio Media Suite software will be available during exhibit hours on April 6-9, 2025, in the Spectra Logic booth (SL8519) at NAB Show, Las Vegas Convention Center, Las Vegas, Nevada. Rio Media Suite software is available for Q2 delivery.
…
Starfish Storage, which provides metadata-driven unstructured data management, is being used at Harvard’s Faculty of Arts and Sciences Research Computing group to manage more than 60 PB involving over 10 billion files across 600 labs and 4,000 users. In year one it delivered $500,000 in recovered chargeback, year two hit $1.5 million, and it’s on track for $2.5 million in year three. It also identified 20 PB of reclaimable storage, with researchers actively deleting what they no longer need. Starfish picked up a 2025 Data Breakthrough Award for this work in the education category.
…
Decentralized (Web3) storage supplier Storj announced a macOS client for its new Object Mount product. It joins the Windows client announced in Q4 2024 and the Linux client, launched in 2022. Object Mount delivers “highly responsive, POSIX-compliant file system access to content residing in cloud or on-premise object storage platforms, without changing the data format.” Creative professionals can instantly access content on any S3-compatible or blob object storage service, as if they were working with familiar file storage systems. Object Mount is available for users of any cloud platform or on-premise object storage vendor. It is universally compatible and does not require any data migration or format conversion.
…
Media-centric shared storage supplier Symply is partnering with DigitalGlue to integrate “DigitalGlue’s creative.space software with Symply’s high-performance Workspace XE hardware, delivering a scalable and efficient hybrid storage solution tailored to the needs of modern content creators. Whether for small post-production teams or large-scale enterprise environments, the joint solution ensures seamless workflow integration, enhanced performance, and simplified management.”
…
An announcement from DDN’s Tintri subsidiary says: “Tintri, leading provider of the world’s only workload-aware, AI-powered data management solutions, announced that it has been selected as the winner of the ‘Overall Data Storage Company of the Year’ award in the sixth annual Data Breakthrough Awards program conducted by Data Breakthrough, an independent market intelligence organization that recognizes the top companies, technologies and products in the global data technology market today.”
We looked into the Data Breakthrough Awards program. There are several categories in these awards with multiple sub-category winners in each category: Data Management (13), Data Observability (4), Data Analytics (10), Business Intelligence (4), Compute and Infrastructure (6), Data Privacy and Security (5), Open Source (4), Data Integration and Warehousing (5), Hardware (4), Data Storage (6), Data Ops (3), Industry Applications (14) and Industry Leadership (11). That’s a whopping 89 winners.
In the Data Management category we find 13 winners with DataBee the “Solution of the Year” and VAST Data the “Company of the Year.” Couchbase is the “Platform of the Year” and Grax picks up the “Innovation of the Year” award:
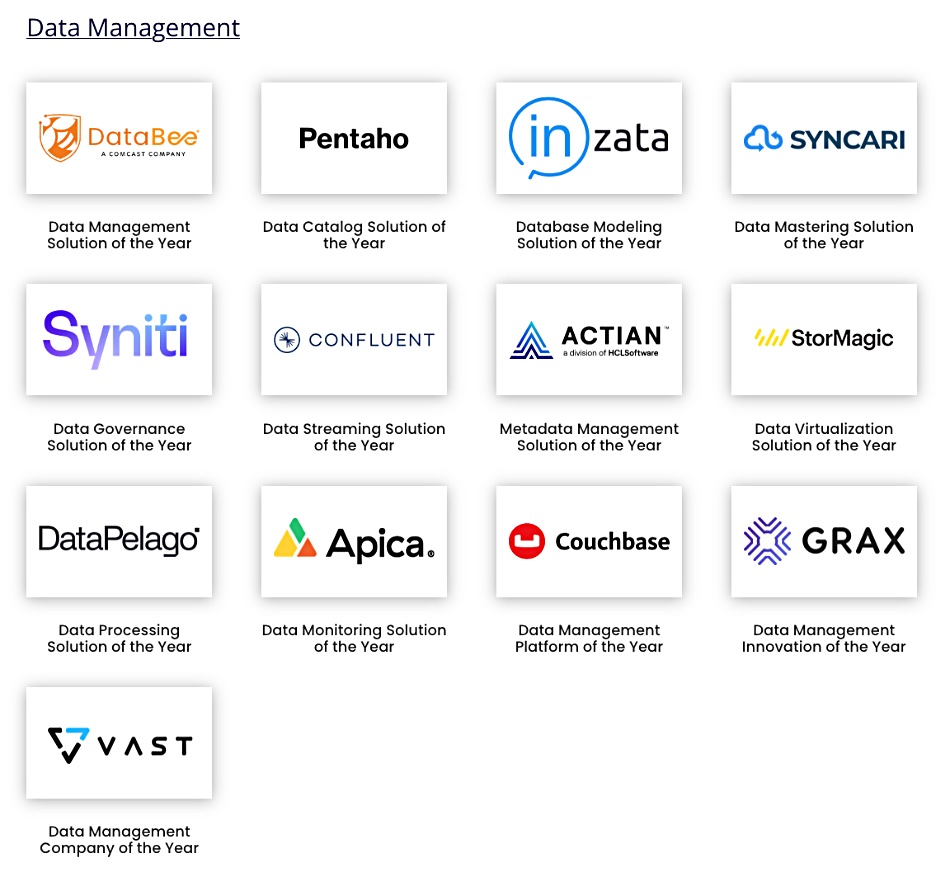
The six Data Storage category winners are:
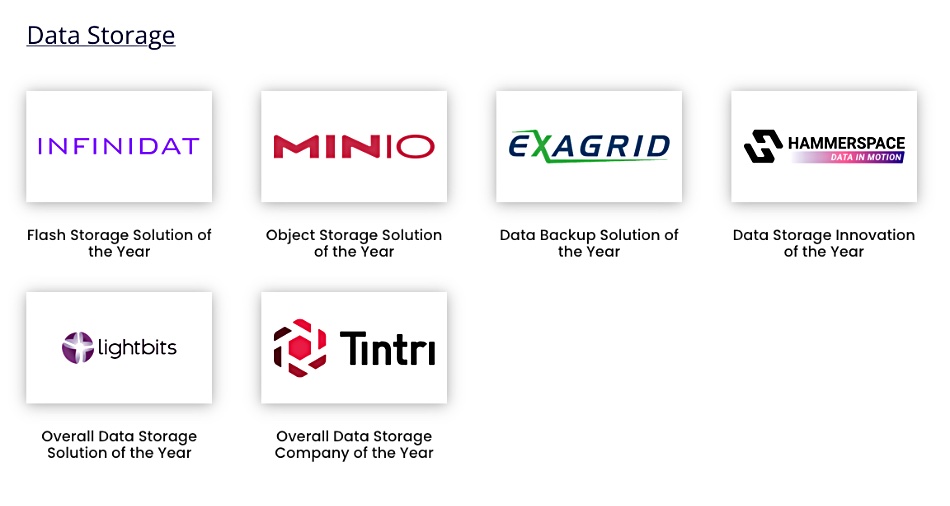
The award structure may strike some as unusual. The judging process details can be found here.
…
Cloud, disaster recovery, and backup specialist virtualDCS has announced a new senior leadership team as it enters a new growth phase after investment from private equity firm MonacoSol, which was announced last week. Alex Wilmot steps in as CEO, succeeding original founder Richard May, who moves into a new role as product development director. Co-founder Dan Nichols returns as CTO, while former CTO John Murray transitions to solutions director. Kieran Brady also joins as chief revenue officer (CRO) to drive the company’s next stage of expansion.
…
S3 cheaper-than-AWS cloud storage supplier Wasabi has achieved Federal Risk and Authorization Management Program (FedRAMP) Ready status, and announced its cloud storage service for the US Federal Government. Wasabi is now one step closer to full FedRAMP authorization, which will allow more Government entities to use its cloud storage service.
…
Software RAID supplier Xinnor announced successful compatibility testing of an HA multi-node cluster system combining its xiRAID Classic 4.2 software and the Ingrasys ES2000 Ethernet-attached Bunch of Flash (EBOF) platform. It supports up to 24 hot-swap NVME SSDs and is compatible with Pacemaker-based HA clusters. Xinnor plans to fully support multi-node clusters based on Ingrasys EBOFs in upcoming xiRAID releases. Get a full PDF tech brief here.