Blocks & Files interviewed Denise Natali, the new VP for Americas sales at Datadobi, over email about the firm’s views on unstructured data management. Datadobi provides a StorageMAP facility to locate, identify and manage unstructured data, building on its core migration technology.
We wanted to gain a better understanding of Datadobi’s position on data tiering, orchestration, supplying a product vs a service, and Gen AI needs. We outlined the context in a basic diagram we sent over:
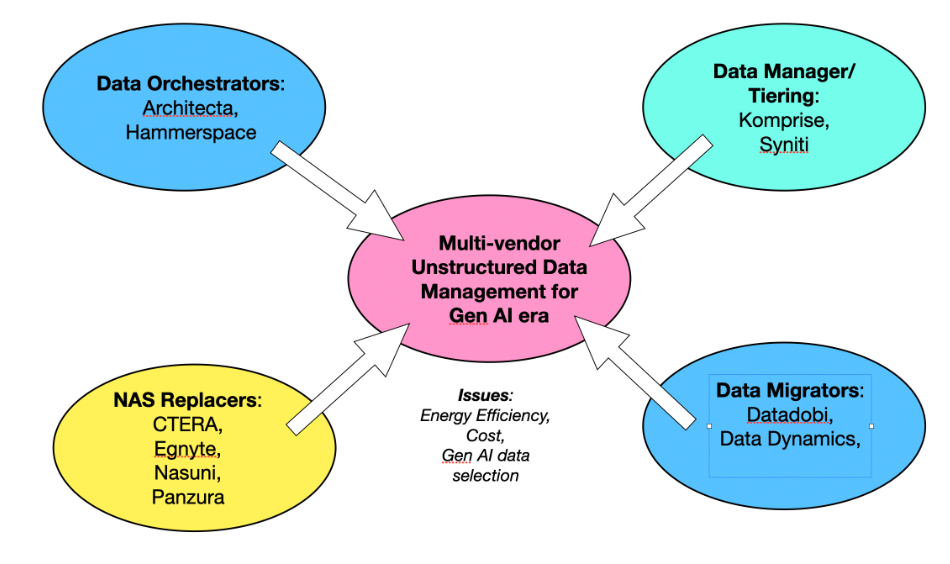
Datadobi reciprocated with its own view of the unstructured data management market:
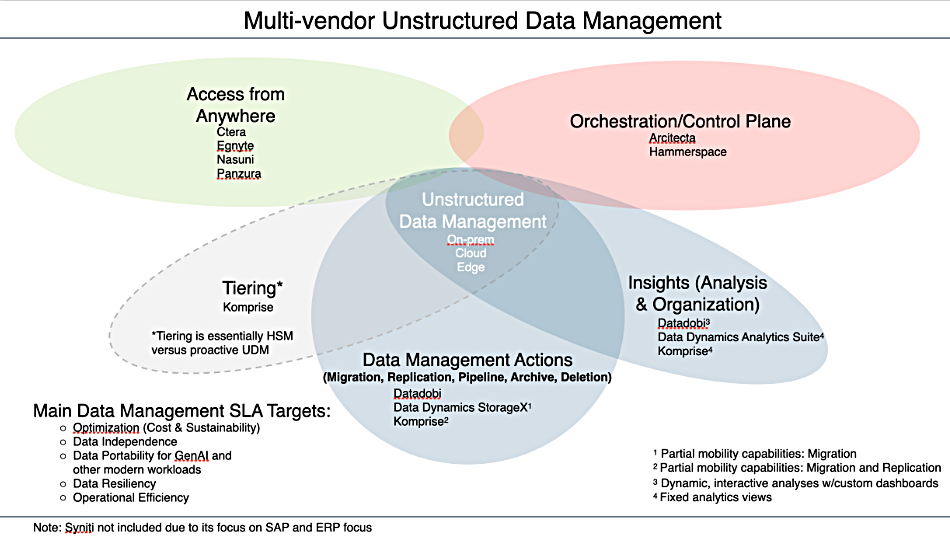
It also sent a note explaining the terms:
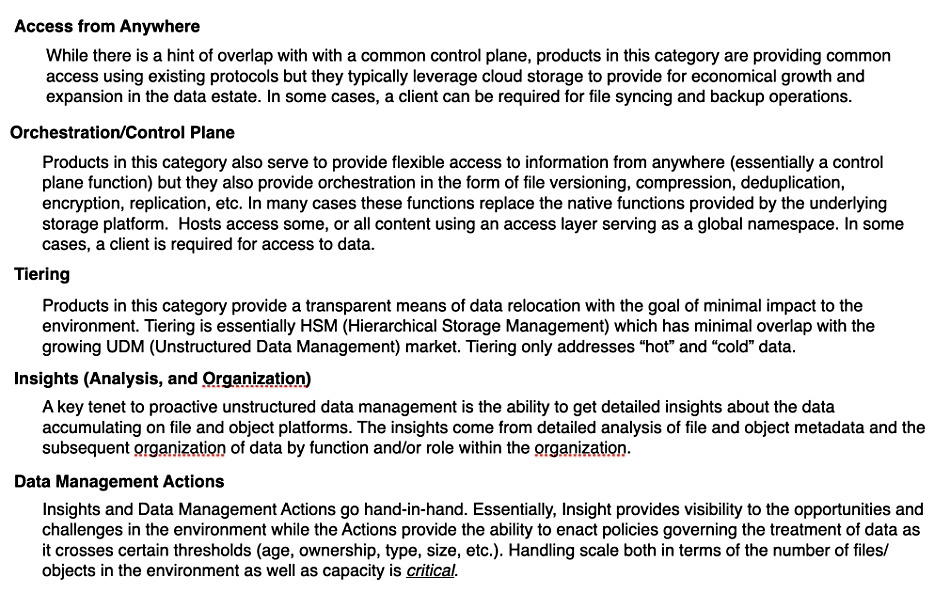
That provides the background context for Natali’s answers to our questions.
Blocks & Files: What is Datadobi’s strategy for growing sales in this market?
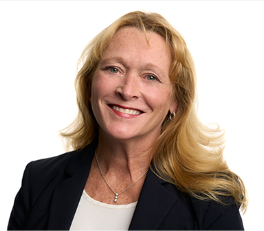
Denise Natali: Priority number one for Datadobi is to stay close to its customers and partners with whom we have such close and trusted relationships. We spend the majority of our time with customers working to understand their needs – we are dedicated to understanding their unique “why?” Only then do we help guide them towards the next steps in the unstructured data management journey – and share how StorageMAP can help them solve specific unstructured data management challenges and achieve desired outcomes.
In addition, we are dedicated to not only staying on top of, but in front of, evolving market demands/trends. So in addition to the time we spend with our customers and partners, there are folks in our organization that spend a tremendous amount of time with industry experts (such as leading industry analysts) and by reading and keeping up to date with business and technology journals (such as yours).
Drilling down a bit … StorageMAP is a versatile solution – and the market needs to know this. But, at the same time, we need to be able to demonstrate our strengths in particular areas – our ability to help our customers and partners overcome challenges and achieve their goals. For instance, one such area of focus is hybrid cloud data services. From a sales standpoint, we need to be able to prove that StorageMAP is hands-down the most robust and comprehensive solution here – a truly vendor-neutral solution, with unmatched unstructured data insight, organization, and mobility capabilities and the only solution capable of scaling to the requirements of large enterprises.
And last but certainly not least, it is critical to our sales process that we demonstrate that StorageMAP enables its customers to maintain data ownership and control – addressing customer concerns about metadata security and compliance, whether the data is managed on-premises, remotely, or in the cloud.
Blocks & Files: Does Datadobi have a data orchestration strategy?
Denise Natali: For sure. Our goal is to always remain at the forefront of unstructured data management technology. Customers and partners want increased automation and policy-driven data management capabilities – so data orchestration is an integral part of our near-term roadmap.
As you likely know, today customers are seeking solutions with data orchestration capabilities for a number of reasons – such as improved data management, enhanced data quality and consistency, increased operational efficiency, better decision making, scalability, compliance and security, and cost savings.
Blocks & Files: Do customers want unstructured data management as a service or as a software product they buy?
Denise Natali: As with any other solution, enterprises are looking at a number of options for delivering unstructured data management. But before we get into that, it is important to note that unstructured data management as a market is still nascent. Right now, we find that our conversations with customers are really focused on their specific and immediate needs and not necessarily a well-defined need either. We take a consultative approach to help them to explore what it is they are trying to achieve, and help them to make that a success. As our CEO likes to say, we deliver an outcome not a software product. That is what our second-to-none reputation is built upon.
Ultimately, enterprises will want an unstructured data management solution to play well with their existing infrastructure. The last thing most of them are looking for is yet another standalone point software [product] that they have to manage. Integration with their ecosystems, whether managed by them or an external party will be key.
But what is essential to all our enterprise customers, whichever path they chose, is that they maintain ownership and control over their data and metadata to make sure it remains secure and compliant with their internal policies and regulatory requirements.
At the end of the day, maintaining ownership and control is exactly why our customers prefer an on-prem solution. Many aaS offerings create security headaches for customers as they lose control of their data. At the behest of our customers, we are exploring various consumption models which are different than aaS models – the choices are not just “purchase/subscribe” vs “aaS”.
Blocks & Files: Does an unstructured data manager have to support all data silos, both on-premises and in the public cloud?
Denise Natali: Absolutely. An unstructured data manager must support all data silos – both on-premises and in the cloud. Here’s why:
1. Hybrid Cloud Strategies – Many organizations adopt hybrid cloud strategies, maintaining a mix of on-premises and cloud-based data storage to optimize performance, cost, and security. An effective unstructured data manager must seamlessly manage data across these diverse environments.
2. Data Mobility – As organizations grow and evolve, the need to constantly move data between on-premises systems and various cloud platforms increases. Supporting all data silos makes sure that the data owners and consumers (whether people or applications such as Gen AI) can easily and quickly get the data where they need it. Liberating data from the confines of specific hardware is key to a successful hybrid-cloud strategy.
3. Unified Management – To streamline operations and reduce complexity, organizations prefer a single pane of glass for managing their unstructured data. A unified data manager that supports all silos provides centralized control and visibility, enhancing operational efficiency. This doesn’t mean that an unstructured data manager (likely in IT) will need to do all the work, but a single point of coordination between the data custodians, data owners, and data consumers, will be vital.
4. Data Lifecycle Management – Different regulatory requirements may apply to data stored on-premises versus in the cloud. A comprehensive data manager can help enforce consistent compliance and other policies across all storage locations through the implementation and monitoring of policies created by the data owners.
5. Optimized Storage Utilization – Organizations can optimize storage costs and performance by strategically placing unstructured data based on usage patterns and access requirements. Supporting all data silos allows for intelligent data tiering and lifecycle management.
6. Scalability and Flexibility – Businesses need the flexibility to scale their storage solutions as needed. An unstructured data manager that supports both on-premises and cloud environments can easily adapt to changing storage demands as they evolve.
Blocks & Files: How does an unstructured data manager support customers adopting Gen AI technology, for training and for inferencing?
Denise Natali: The ideal unstructured data manager can support customers adopting Generative AI technology for training and inferencing through several key functions. It is important to note here that when Gen AI applications (and many other kinds of applications) claim to process “unstructured data” what they mean is they can deal with small amounts of unstructured data. Ten terabytes of unstructured data is likely considered to be fairly large for most applications.
What StorageMAP can help with is identifying the right 10TB from the multiple petabytes and billions of files that enterprises have at their disposal, making sure that only high-quality and pertinent data is used for AI model training.
As a side note, applications that perform other tasks – such as looking for PII data within a file – have the same limitation. StorageMAP does not replace these applications, but can make them far more effective by helping to get the right data to them rather than the current approach of “best guess.”
****
By adding data orchestration functionality Datadobi will be competing with Architecta and Hammerspace, and able to upsell in its existing customer base..