We asked HPE to summarise its data storage product positioning to help us prep a recent interview with HPE Storage boss Tom Black.
In addition, we emailed the company some questions about its storage strategy and here are the answers provided by Tom Black’s staff. The Q&A is lightly edited for brevity and is also available as a PDF. (The document also contain’s HPE’s summary of its storage product line-up, which comrpises the Intelligent Data Platform, InfoSight, Primera, Nimble Storage, XP, MSA SAN Storage, Nimble Storage hHCI, SimpliVity, Cloud Volumes and Apollo 4000.)
Blocks & Files: Does Tom Black look after HPC storage? I’m thinking of the ClusterStor arrays and DataWarp Accelerator that were acquired when HPE bought Cray. If not will there be separate development or some sort of cross-pollination?
HPE: No, HPC and HPC storage are part of an integrated group in a separate business unit. HPC is run as a segment with integrated R&D and GTM.
Blocks & Files: IBM and Dell are converging their mid-range storage products. Does HPE think such convergence is needed by its customers and why or why not? Will we see, for example, a converged Primera/3PAR offering and even a converged Primera/3PAR/ Nimble offering?
HPE: We believe that businesses need workload-optimized systems to support mid-range and high-end requirements. Systems that are designed to deliver the right performance, resiliency, efficiency, and scale for the SLA and use case.
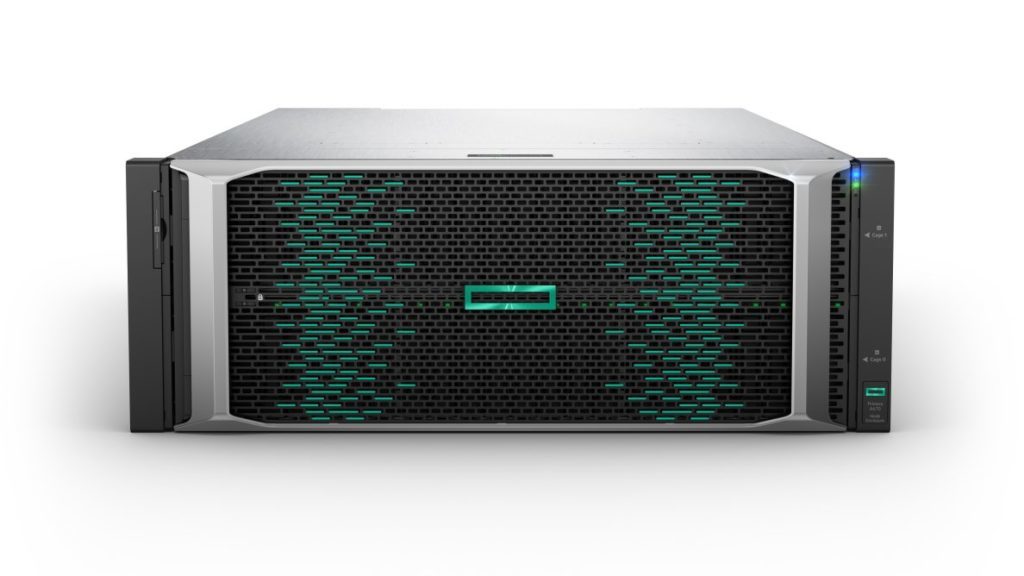
With the introduction of HPE Primera last year, HPE consolidated its primary storage portfolio with one lead-with mid-range storage platform – HPE Nimble Storage, and one lead-with high-end storage platform – HPE Primera. Our HPE 3PAR installed base has a seamless upgrade path to HPE Nimble Storage and HPE Primera. (Comment: We didn’t know that 3PAR arrays could be upgraded to Nimble arrays.)
Blocks & Files: Will the XP systems remain a key aspect of HPE’s storage portfolio and, if so, how are they distinguished from the Primera line?
HPE: Yes, HPE XP Storage is a key aspect of HPE’s storage portfolio. It is our high-end platform for mission-critical workloads with extreme scale requirements, traditional use cases like Mainframe, NonStop, OPVMS and Cobol-based environments, and XP install base customers. HPE Primera is our lead-with high-end platform for mission-critical applications to support virtualized, bare metal, and containerized applications.
Blocks & Files: I believe HPE is the only supplier with both HCI and dHCI systems in its range. How does HP position its SAN storage, its HCI systems and its dHCI systems?
HPE: In the last several years, HCI has offered small and medium businesses a new value proposition, delivering infrastructure simplicity with VM-centric operations and automation – and therefore HCI (and HPE SimpliVity) is performing very well in that segment.
We’re hearing from our enterprise and mid-market customers that they want the simplicity of HCI but are unable to adopt it as they’re giving up too much to gain the agility and simplicity. This is where we position HPE Nimble Storage dHCI – a disaggregated HCI platform that uniquely delivers the HCI experience but with better performance, availability, and scaling flexibility than HCI for business-critical applications and mixed-workloads at scale.
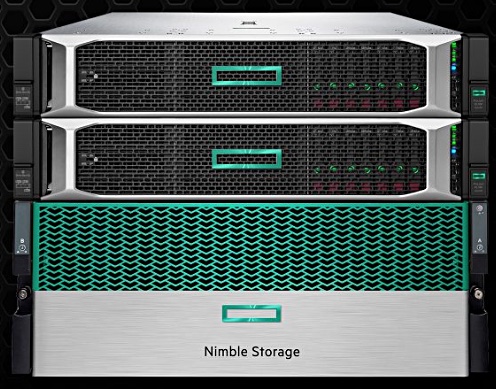
In addition, in the enterprise core data center and service provider market, organizations here have the largest scale requirements and strictest SLA for performance and availability for their mission-critical applications. The right solution for them is high-end storage/SAN, so we position HPE Primera with HPE Synergy here as the core consolidation platform for mixed-workloads at scale.
Blocks & Files: What is the role of storage in HPE’s composable systems? Will all the SAN, HCI and dHCI systems evolve towards composability?
HPE: HPE has been a pioneer in delivering composability. Companies once forced into overprovisioning infrastructure are now able to create fluid pools of compute, storage, and network resources. Composability is designed for any application, at any SLA, and at any scale because all it takes is a single line of code to gather and deploy the IT assets needed to run any application, anywhere, at any scale.
From a storage perspective, our focus is to continue to deliver infrastructure that is fully automated, optimized and integrated with intelligence and composability. We drive a cloud experience on-premises with a strong foundation of composability on intelligent infrastructure for any workload. Bringing together the intelligence from an intelligent data platform with the composability of HPE Synergy, IT organizations have the absolute flexibility to support any application with the agility of the cloud in their on-premises data center—delivered as a service to scale to meet ever-changing business needs.
We fundamentally believe in flexibility and the need for workload-optimized systems designed for the use case. Composability – with the ability to support any applications across bare metal, virtualization, and containers – makes the most sense in large scale, enterprise core data center environments. For organizations who don’t need this level of resource composition and flexibility, and perhaps are exclusively needing to support virtual machines, HCI – with a software-defined framework specifically for virtual machines – will make the most sense for them.
Blocks & Files: HPE is not perceived as a mainstream supplier of its own file storage technology. It partners widely with suppliers such as Qumulo, WekaIO and others. Long term should HPE have its own file storage technology or continue to set as a reseller/system integrator of other supplier’s technology?
HPE: With unstructured data, use cases are continuing to evolve. Here’s the breakdown of our view of use cases and how we address them with our owned IP and partner solutions:
- AI/ML – HPE Ezmeral Container Platform, which is inclusive of HPE Ezmeral Data Fabric (MapR), enables data scientists and data analysts to build and deploy AI/ML pipelines to unlock insights with data.
- Distributed, scale-out enterprise-wide data fabric for edge to cloud – HPE Ezmeral Data Fabric (MapR) is a leading scale-out filesystem with enterprise reliability and data services, and one of the only file systems that offers batch processing, file processing, and streams processing.
- Analytics, Medical Imaging, Video Surveillance, etc – HPE Apollo 4000 in partnership with scale-out software partners such as Qumulo (file) and Scality (object) provides a great foundation for these use cases.
- HPC – We have market-leading HPC solutions (i.e. ClusterStor) from our acquisition of Cray
Like any market, we will continue to monitor the evolution of customer use cases and ensure we can help them address the use cases with differentiated solutions.
Blocks & Files: HPE is not perceived as a mainstream supplier of its own object storage technology. It partners with suppliers such as Cloudian and Scality. Long term should HPE have its own object storage technology or continue to set as a reseller/system integrator of these other supplier’s technology?
HPE: HPE will continue to monitor the market for object storage. Scality is the strategic HPE partner for massively scalable multi-cloud unstructured data stores – including S3 compatible object storage. The HPE partnership with Scality is global and backed by joint go-to-market. This is all in addition to a global reseller agreement through which HPE offers Scality on our mainstream HPE price list.
With unstructured data, use cases are continuing to evolve. Here’s the breakdown of our view of use cases and how we address them with our owned IP and partner solutions:
- AI/ML – HPE Ezmeral Container Platform, which is inclusive of HPE Ezmeral Data Fabric (MapR), enables data scientists and data analysts to build and deploy AI/ML pipelines to unlock insights with data.
- Analytics, Medical Imaging, Video Surveillance, etc – HPE Apollo 4000 in partnership with scale-out software partners such as Qumulo (file) and Scality (object) provides a great foundation for these use cases.
- HPC – We have market-leading HPC solutions (i.e. ClusterStor) from our acquisition of Cray
Like any market, we will continue to monitor the evolution of customer use cases and ensure we can help them address the use cases with differentiated solutions.
Blocks & Files: How will HPE evolve its storage offer at Internet Edge sites with a need, perhaps, for local data analytics?
HPE: Enterprise edge, as we refer to the edge, is becoming more and more strategic for customers and an opportunity for infrastructure modernization. It can be a small site in a hospital or oil rig, or a distributed enterprise with remote offices/branch offices e.g. for banks or retail. Key characteristic is often no on-site resident IT staff and therefore the ability to remotely deploy, manage, operate, upgrade is really important.
We are seeing a growing market opportunity to leverage HCI at the enterprise edge. The simplified experience of a hyperconverged appliance addresses edge-specific requirements, including the need to power edge applications in a highly available, small footprint, protect data across sites, and facilitate entire lifecycle management remotely without local IT presence. HPE SimpliVity is our strategic platform to address this need with customers with numerous use cases and wins.
We also provide our customers the end-to-end lifecycle with HPE Aruba and our HPE EdgeLine servers to service all customer needs at the edge.
Blocks & Files: How is HPE building a hybrid cloud storage portfolio with data movement between and across the on-premises and public cloud environments?
HPE’s approach hinges on providing a true, distributed cloud model and cloud experience for all apps and data, no matter where they exist – at the edge, in a colocation, in the public cloud, and in the data center. Our approach helps customers modernize and transform their applications and unlock value across all stages of their lifecycle where it makes the most sense.
Our hybrid cloud storage portfolio: 1) on-premises private cloud, 2) enterprise data services across both on-premises private cloud and public cloud, and 3) seamless data mobility between on-premises private cloud and public cloud.
HPE delivers a seamless experience by extending our storage platforms to the public cloud with HPE Cloud Volumes. HPE Cloud Volumes is a suite of enterprise cloud data services that enables customers, in minutes, to provision storage on-demand and bridges the divide and breaks down the silos that exist between on-premises and public cloud.
Unlike other cloud approaches, we’re uniquely bringing customers a fresh approach to backup, with the ability to put backup data to work in any cloud with no lock-ins or egress charges when recovering that backup data from HPE Cloud Volumes Backup.
Blocks & Files: How does HPE see the market need for SCM, NVMe-oF, and Kubernetes and container storage developing. Will these three technologies become table stakes for each product in its storage portfolio?
HPE – SCM
HPE 3PAR Storage pioneered the use of SCM, showcasing its performance benefits as early as 2016. Next, in 2019, we were the first vendor to introduce SCM as a caching tier on the 3PAR high-end platforms for extreme low latency requirements.
While other storage vendors have since caught up, SCM remains a niche use case because it is cost prohibitive to deploy as a persistent storage tier.
Our focus has been to democratize SCM and delivering it more widely through broader use cases. This is why this past June we introduced SCM for HPE Nimble Storage to deliver SCM-like performance for read-heavy workloads with 2X faster response times with an average of sub 250 microseconds latency at near the price of an all-flash array (~10-15% price premium depending on the HPE Nimble Storage all-flash array model).
Over time, we believe SCM will do the job of what SSD did 10 years ago, by providing a latency differentiation performance tier within AFAs.
HPE -NVMe-oF
We believe this is the next disruption in the datacenter fabrics. As with all fabric changes, this transition will happen gradually. It will take time for host ecosystems readiness, as well as customers’ willingness to make substantial changes in their fabric infrastructure.
We are at the early stages of host ecosystem readiness. For example, Linux distributions are ready. VMware 7.0 has brought some level of fabric support (with the next release scheduled to go further).
For us, we know this disruption is coming, and are working hard with our partners to develop an end to end NVMeoF solution. We are committed to being ready once the market (partners and customers) is as well.
HPE – Kubernetes and Container Storage
As customers continue to modernize their apps, they need to modernize the underlying infrastructure and we see a clear shift toward container based applications. Kubernetes is the defacto open source standard.
HPE CSI Driver for Kubernetes for HPE Primera and HPE Nimble Storage enables persistent storage tier for stateful container-based apps, and for deploying VMs and containers on shared storage infrastructure. HPE CSI Driver for Kubernetes includes automated provisioning and snapshot management. Over time, we will extend CSI (which is an open specification) to deliver additional value-added capabilities for customers.
In addition to HPE Storage platforms for container support, HPE offers its Ezmeral Container Platform, to help customers modernise and deploy non-cloud native and cloud native applications. (See answers above.)