IBM is extending Ceph’s block and file capabilities and positioning it as a backend data store for AI workloads behind its Storage Scale parallel file system.
Ceph is open source scale-out storage software providing file, block, and object interfaces on top of an underlying object store, with self-healing and self-managing characteristics. IBM acquired Ceph when it bought Red Hat for $34 billion in 2019. Just over a year ago it moved the Ceph product from its Red Hat business into its storage organization and rebranded it as Storage Ceph. We found out a little about its plans for Ceph from a blog written by Gerald Sternagl, IBM Storage Ceph Technical Product Manager, last month. Now a briefing from IBM Storage General Manager Denis Kennelly has revealed more.
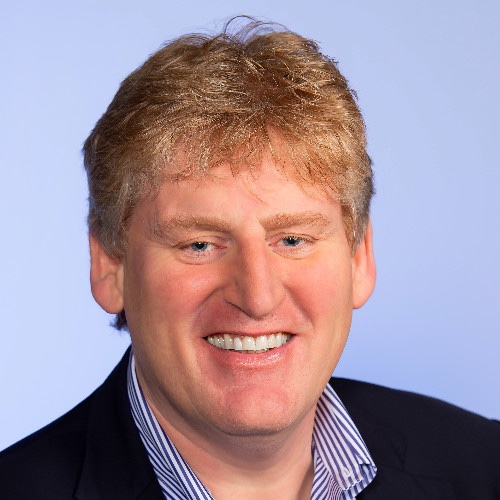
He is responsible for all IBM storage, both hardware and software products, with the approximate revenue split being two thirds hardware and one third software. Kennelly said IBM grew its hardware storage market share in 2023, mentioning the high-end DS8000 arrays and FlashSystem all-flash arrays. Are Ceph sales growing? “Yes, absolutely,” he says.
There are three focus areas for IBM Storage: hybrid cloud, AI, and data recovery and resilience. Ceph has a role in hybrid cloud and AI, where it helps deliver access to unstructured data before it is brought to large language model processing systems.
IBM’s position in the data recovery and resilience area is helped by the Cohesity-Veritas acquisition as its Storage Defender product involves a partnership with Cohesity. The deal, in principle, will aid selling to the Veritas customer base. In Kennelly’s view: “Consolidation is now clearly happening in the backup market.”
Back to Ceph. Kennelly sees Ceph as fitting the market need for software-defined storage, saying: “Red Hat closely coupled Ceph, OpenShift and containers. We want to accelerate that with a full software-defined storage stack running on commodity hardware.” We’re thinking server/storage hardware from Dell, HPE Lenovo, Supermicro etc.
In the past year IBM has added NVMe/TCP support to extend Ceph’s block storage capability, and also improved its usability. He suggested that when a 100 TB storage capability was needed for an AI project, it’s hard work with a SAN. Extending it can take 20-30 individual steps.
“In the Ceph world you roll in another 100 TB in a box, add it to the cluster, and off you go.” Ceph will automatically be able to use that. He added: “The WatsonX team are working closely with Ceph.” WatsonX being IBM’s generative AI platform.
Is IBM considering adding at adding GPUDirect support to Ceph? “It’s something we’re looking at,” he said, although IBM Storage has great software to deliver data fast to GPU servers via GPUDirect – and that’s Storage Scale with its parallel file system.
Kennelly said: “Storage Scale has GPUDirect. We use Scale to do that with Ceph underneath it.”
Scale has its AFM (active file management) scalable, high-performance, file system caching layer that can link to a Ceph backend. AFM enables users to create associations from a local Scale cluster to separate storage or a remote cluster, and define the location and flow of file data to automate the data’s management. You can implement a single namespace view across sites around the world.
IBM has been carrying out Storage Scale benchmarks and Kennelly is very happy with them. We can expect published results later this year. The Scale-Ceph idea is to leave the data where it is and IBM will query it. This is different from new vendors such as Snowflake and Databricks who say: ”Bring us the data and we’ll query it.”
Kennelly said: “We need to do a fast query and that’s why we use Storage Scale. You can pick straight NFS but you’ll never get the performance.”
In his view: “It’s an exciting time. We’ve a lot to get done. AI will be quite transformative.”
Ceph will have a strong base platform role in this by acting as an underlying data store with Storage Scale using Ceph-held data to feed to LLM models in GPU servers.