Silverton Consulting’s president, Ray Lucchesi, has looked at the MLPerf storage benchmark’s initial results and suggested that simulated accelerators per compute node is a useful way to compare suppliers.
Last month we looked at this first MLPerf storage benchmark, which examines how well storage systems serve data to simulated Nvidia V100 GPUs. We focused on the MBps values per supplier system and found not that much difference between suppliers. DDN suggested we should focus on how many GPUs (accelerators in MLPerf terminology) are driven at a specified performance level from a given number of controllers, number of SSDs, and how much power is needed. DDN drives 40 GPUs from a single AI400X2 (2 controllers) and Weka drove 20 GPUs from 8 nodes. Nutanix agreed with this accelerator number per system metric, and it could drive 65 accelerators with a 5-node Nutanix cluster, 15 accelerators per node.
Lucchesi observed: “What they are showing with their benchmark is a compute system (with GPUs) reading data directly from a storage system.” We should use the number of simulated accelerators as a relative number to compare suppliers’ system and not an absolute number of V100 GPUs supported as it’s a simulated and not physical accelerator. A constraint of the benchmark is that storage systems should keep the simulated V100 90 percent busy.
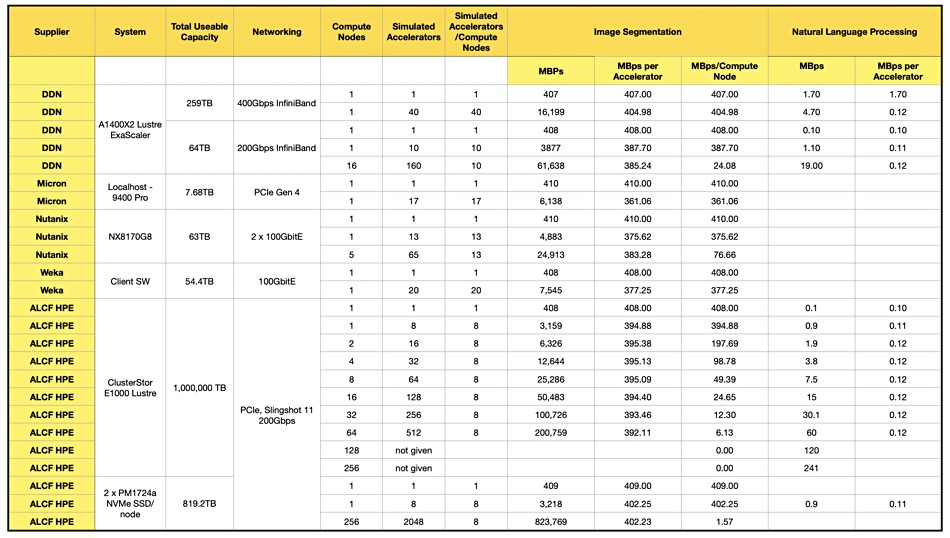
Lucchesi inspected the results and noted a disparity with, for example, DDN. One of its results, 160 accelerators with 64TB of flash storage, listed 16 compute nodes, while another for 40 accelerators listed just one compute node but had 259 TB of flash – more capacity but a lower result. This is counter-intuitive. Lucchesi suggests “the number of compute nodes makes a significant difference in simulated GPUs supported.”
We added a simulated accelerators over compute node column for image segmentation to our table of MLPerf supplier data, as above, and then charted the result:
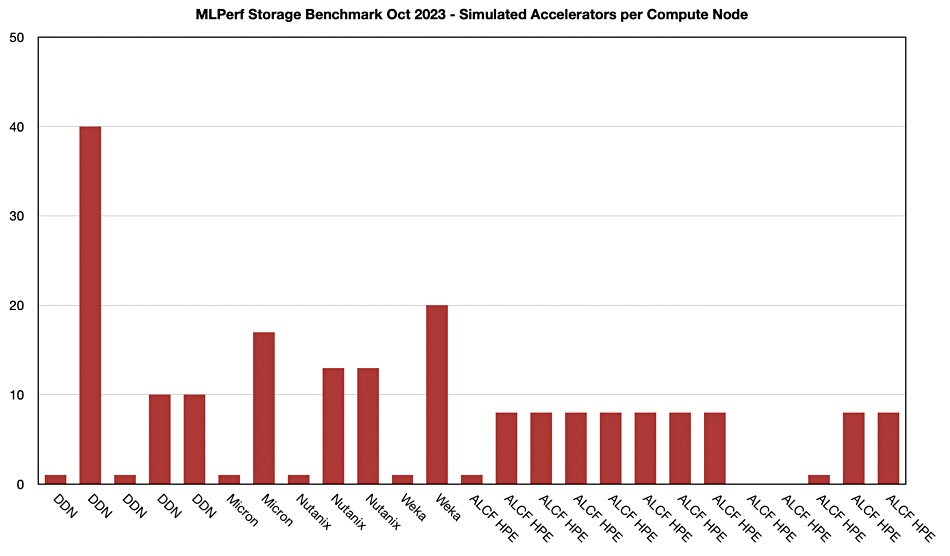
The highest result per supplier – meaning DDN, Weka, Micron, Nutanix, and HPE – are nicely differentiated from each other.
Lucchesi writes: “The Micron SSD used an NVMe (PCIe Gen4) interface while the other two storage systems used 400 Gbps InfiniBand and 100 Gbps Ethernet, respectively. This tells us that interface speed, while it may matter at some point, doesn’t play a significant role in determining the # simulated V100s.”
He suggests ways in which the benchmark could be improved to facilitate better comparisons between suppliers:
- There should be different classes of submissions, with one class for only 1 compute node and another for any number of compute nodes.
- He would uprate the simulated GPU configurations to A100 rather than V100s, one generation behind best-in-class GPUs.
- Have a standard definition for a compute node – the compute node hardware/software should be locked down across submissions.
- Clearly specify the protocol used to access the storage.
- Describe the storage configurations with more detail, especially for software-defined storage systems.