Interview: Compared to file, object has simply become a protocol absorbed into an analysis solution, and proprietary GPUDirect will be followed by more generic multi-GPU-vendor technology. These are points made by Qumulo CTO Kiran Bhageshpur when asked about the relative status of object and file storage on the one hand and the role of GPUDirect and object storage on the other. Qumulo supplies scalable and parallel access file system software and services for datacenters, edge sites, and the cloud with a scale anywhere philosophy. It supports the S3 protocol with Qumulo Shift, but not GPUDirect.
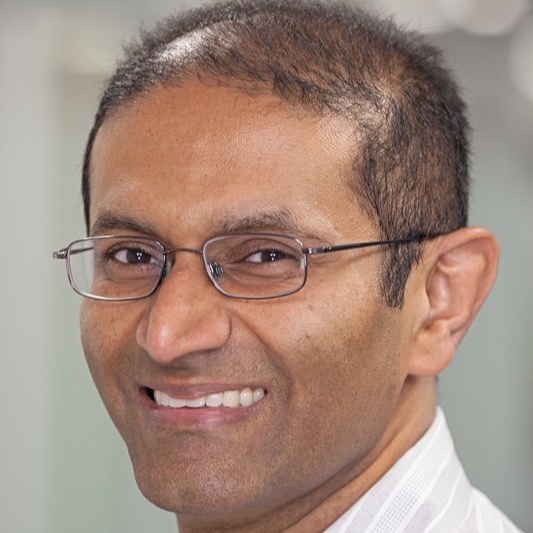
The surge of interest in AI means Qumulo is placed at the intersection of large unstructured data sets, GPU processing, and LLM training and inference. Nvidia’s GPUs dominate the LLM training market and there are two distinct populations of unstructured data – files and objects – on which these GPUs can feed. How does Bhageshpur view their relative status?
He said: “My firmly held opinion is that object in the cloud is an absolute first-class citizen … It is a fundamental primitive; S3 and EC2 and EBS were the first three services as a part of AWS back in 2006. And it is insanely durable. It is ridiculously available. It is got fantastic throughput characteristics, not latency, but throughput. So therefore, it is impossible to overwhelm object in the cloud. It’s got a huge scale. They talk about zone-redundant storage, they talk about locally redundant storage. You could do replication as a part of the setup to a different region. It’s fantastic.”
But “object on-premises is a cruel joke.”
Qumulo supports the S3 protocol “simply because we support a protocol.”
On-premises object storage is a pale imitation of in-cloud object storage, he says. “Nobody has the capability to actually deliver that level of redundancy that exists in the cloud on-premises. And even if they do, it is tiny in terms of the number of people who can operationalize it. Think about zone redundant storage which is an S3 basic characteristics, which means they have three datacenters, all of them with separate power cooling, networking, and yet only one millisecond to one and a half millisecond latency between these datacenters. And they are essentially doing erasure encoding of the object across these things. How many people on earth do you think outside the magnificent seven, and maybe another 10 to 12, can actually do this?”
The magnificent seven refers to Amazon Web Services (AWS), Microsoft Azure, Google Cloud Platform (GCP), Alibaba Cloud, IBM Cloud, Oracle Cloud, and Salesforce.
General enterprise datacenters may have “three datacenters in three different states. Now your latencies are 30, 40, 50, 60, 70 milliseconds; the experience is crappy. And now you get the sort of opinion that it is slow.”
But “then you will have people like Pure and VAST come along and say, hey, it’s fast object. But, if you look at the use cases, it’s not quite there. Now, let me flip that around to something else and talk about file.”
“Enterprise file, whether it is Dell EMC Isilon, or Qumulo or NetApp, is world class. It is fantastic. It is scalable, it’s reliable, it is affordable. It’s great. I’ll even throw in VAST and give them credit; it’s really good service on premises.”
However, he says, “file in the cloud … is a bit of a cruel joke, until we came along with Azure Native Qumulo.”
“Think about it. EFS is just NFS highly scalable, good shared storage. But it’s got none of the features, it’s got no … quotas and replication and snapshots … Azure NetApp Files is actually hardware in the cloud datacenter … If you look at all of these, they are 10 to 20 times more expensive than what is available on-premises.”
“Look at the price point on, I just pick on Azure NetApp. The premium tier, the ultra premium fast thing on Azure NetApp Files, is over $300 per terabyte per month. This is publicly available.”
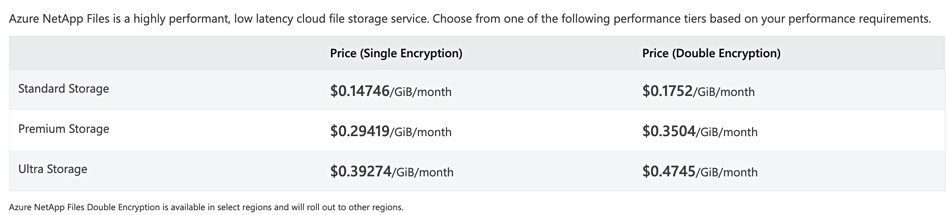
Bhageshpur asserts: “It still is a robust business … And that’s because it is speaking to a palpable need for file. So the whole thought process that file is going to die because of object was nonsensical, and everybody is recognizing that including the cloud guys.”
“That’s why people like Microsoft have partnered very closely with us with Azure Native Qumulo as a service out there. And if you think about it, it’s going to have all the performance of file on premises, it’s got all the elasticity of the cloud in every possible way, when you’re paying for only consumed services, whether it is performance or capacity. So you’ve got the best of both worlds.”
“One of my one of my colleagues, who spent a lot of time at Dell, and AWS, and now is with us running our file business, Brandon Whitelaw, loves to say that, if you take any of the file services in the cloud, wrap, you know, sheet metal around that and try to sell it to an on-premises buyer, they will laugh at that, because it’s so not better from the features, functionality, cost point of view.”
In his view: “When we bring in a unified service, which has got the best of both worlds, all the power and POSIX compliance of file with the cost elasticity of object, that’s where it changes the game. That’s my take on that one.”
“There are niche use cases for object on-premises, such as being an S3 backup target for Veeam or Rubrik, running a Kafka workflow or a … Spark workflow on-premises in conjunction with the cloud offering.”
But object on-premises has not had a great run. “Nobody has had a big outcome … There’s no big standalone object company. Is it Scality? Is it Cloudian? MinIO has come along with an open source plane, that’s kind of clever … In fact, object has simply become a protocol absorbed into an analysis solution … We have object, Pure supports object, VAST supports object. It is just a protocol that we think about. It’s a standard version of an HTTP protocol. That’s all, OK?”
In summary, object on-premises is a cruel joke and basically just protocol support. File in the cloud is a cruel joke, apart from Azure Native Qumulo.
GPUs and GPUDirect
We discussed sending unstructured data to GPU servers, specifically Nvidia GPUs. Unstructured data lives basically in file or object repositories. Nvidia has its proprietary GPUDirect protocol for getting NVMe SSD-held file information to its servers direct from storage with no time-consuming host server CPU intervention. Can we envisage a GPUDirect-type arrangement for objects? Bhageshpur argues that we should not consider developing such a protocol.
“Let me articulate what I think is happening, right? Nvidia is the sexiest company on the face of this planet. Today, it’s joined Amazon and Apple and Microsoft, in being one.”
“GPUDirect is not a technically complicated thing. Especially for a product like Qumulo, which is a user space protocol running on the richness of file. Supporting RDMA is trivial to go do, right? But also, the thing that you gotta go ask yourself, and we ask ourselves this very, very seriously all the time, is: what is the problem? And for which customers?”
“GPUDirect is an Nvidia thing. Does that work with Intel’s Gaudi 2 architecture? No. Does that work with the Microsoft Maia chipset coming out? What happens when there is the next-generation silicon in AWS?”
In other words, he appears to be saying, GPUDirect and Mellanox Ethernet NICs are proprietary lock-in technologies.
Bhageshpur asks: “What’s the generic solution? To get data, independent of file, object or whatever else out there? And what that is is the generic problem to solve.”
He admits: “There are a modest number of players on-premises inside the Nvidia GPU infrastructure, which have just built out massive amounts of Nvidia GPU farms and they probably have GPUDirect storage, whether it is NetApp, or Pure, or our Dell or Vast behind that … And there are small numbers of very large customers.”
But this is not general across the market, although big numbers are involved. ” The reality for AI in general is I’ve talked to a lot of folks [and] by and large, broadly, it’s very much in the experimental stage. I mean, ChatGPT gets our attention, Google Bard gets our attention … ServiceNow announced that it’s had a billion dollars in additional revenue in the last one year because of their AI integration.”
The use case is almost trivial in one way. “If you look at what they have done, it’s pretty straightforward. It is a modern version of a better chatbot in front of it, right? … You’re going to do the things in your workflow engine that you would otherwise have very complex commands and connect things up [to do]. But that’s powerful. That’s really useful.”
However: “It’s in a highly experimental stage. We find a lot of the experimentation is going on either at small scale at a desktop level … or it’s happening in the cloud, not counting the small number of people who do have large farms out there.”
Bhageshpur notes: “It’s not very obvious what the thing is, and whether there is actually going to be everybody doing the training. Is training going to be something that; pick a generic Fortune 1000 company does, or do they consume the output of that as a package service? I think the jury’s still out.”
While the jury is still out, “our focus is really about data and scaling anywhere. Do we have the solution for our customers on premises on any of their infrastructure? Do they get the exact same file and data services in the cloud, any of the clouds that they may choose to go?What about out at the edge [where] they have completely different requirements? Can they exist and operate a single data plane across this, both from a control as well as data visibility and data access point of view?”
Focusing on this, he says: “From a data point of view, getting that to be a really robust solution across, is going to allow us as a company to intersect what the AI trend lines do, no matter which way it goes.”
This is because “inference at the HQ, you have a solution out there. Training in the datacenter, you got a solution out there. You’re gonna go training and inference in the cloud, you got a solution for data out there. How do you connect the GPUs to the data no matter where it is, is the problem we want to solve rather than a point solution.”
He wants us to realize, though, that GPUDirect (NFS over RDMA) has validity. “Don’t get me wrong. That’s not hard to do. And it’s not unreasonable for us to do it. But it’s also, I know, there’s so much talk about it that everybody thinks that’s the cat’s meow and that’s the thing which is most magically required. And it’s an important, but not indispensable, part of what strategy you should be [looking at] going forward.”
Our sense is that Qumulo would like there to be fast file data access technology covering GPUs in general – Nvidia, Intel, AMD, Microsoft, Amazon, and Google ones. And it thinks supplying file data fast for LLM training and inference in the public cloud, at edge sites and in datacenters is also going to be needed.
We think that, until the GPU hardware players come up with a standard interface, a kind of multi-vendor GPUDirect that can be used to put data in any GPU’s memory, then supporting very large GPU players with supplier technology-specific software will be largely inevitable. It is not in Nvidia’s interest to enable GPUDirect-type support for Intel’s Gaudi 2, AMD Radeon or Microsoft’s Maia chips.
GPUDirect and S3
But could we have a GPUDirect for S3? Bhageshpur thinks not. “There is no such thing as S3 over RDMA, because, essentially, GPUDirect is NFS over RDMA.”
Also, the big CSPs face another problem here. He says: “Let me talk about one more reason why RDMA is over bought. You need network card support to make that happen, right? It’s not generic on anything. You need a the network interface to be able to support RDMA to be able to go make it work.” He rhetorically asks: “Guess which is the most prominent networking card which supports RDMA? Do you want to take a guess on which one it is?”
I said Ethernet ones and he almost pounced on this answer: “Who makes them? Mellanox. And who owns Mellanox? Nvidia.”
“If you look at the clouds, various clouds in various regions have pockets where they have Mellanox interfaces on their CPU side that you can go leverage. But it’s various pockets, and it’s kind of scattered; it’s not standard. You’re not going to see Mellanox type RDMA-enabled Ethernet cards ubiquitously available in the cloud for a long time … We know that getting into every other place means that these guys have to go through a sort of hardware refresh cycle, which takes years, you know, to have ubiquitous availability.”
“There are going to emerge different technologies other than GPUDirect, especially in the cloud, to bring data faster from where it lives closer to the GPU.” He wouldn’t be drawn on any details about this.
Bhageshpur thinks that the compound effect of individual productivity enhancements from AI could be huge.
“The real thing to think about is how many people are going to train foundational models? I would argue it is extremely small. How many people are going to update foundational models with specific data? I would think that is also small. It’s not going to be hundreds of thousands. And then how many people are going to consume that? It’s a lot, right?”
“One analogy I’ll make is, how many people build their own distributed file system? If you’re a company, not too many. You look to somebody else to solve that infrastructure problem, because it’s hard. It’s kind of the same thing with foundational models. Training foundational models is difficult … How is the generic enterprise going to go update that?”
He doesn’t think it will, and the main use for AI LLMs is “all about individual productivity, which is why, if you look at the focus, the big part of Azure is a Copilot for everything, which is: let us use our technology to make an individual inside a corporation more productive.”
He says: “That’s exactly what ServiceNow has also done. And by the way, to some extent, maybe it’s correlation and not causation, but it shows up in terms of the US, which is the dominant adopter of all of these things, having had this huge productivity spike in the last 18 to 24 months.”
The answer to the GenAI and ChatGPT question “isn’t going to be as simple and as reductionist as, oh my God, AIG and Terminator, and Skynet … That’s a joke, it doesn’t work. It’s going to be really compelling in mundane ways. It is going to make everybody a little bit more productive.”
“If you’re seeing 5, 10, 20 percent improvement in productivity among your workforce, that’s huge. And people are willing to pay for that.”
And Qumulo’s function? “All the focus in our tiny, teeny little role here is: how do we help our customers who have all of that data stored on us to go take advantage of the [AI] services, build the appropriate connection such that the jobs are done much easier.”