Quantum is developing AI software that can inspect unstructured data stored in its StorNext file system and ActiveScale object storage to identify content in videos, images and documents.
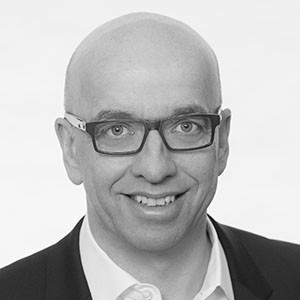
The extent of this was revealed in an interview with Quantum’s technical director for AI and Cloud, Plamen Minev, published in Authority magazine. Minev works in the Quantum CTO office.
Quantum has already developed its AI and ML Content Enhancement Solution powered by the CatDV media asset management system and StorNext file management. It integrates Nvidia DeepStream, Riva, and Maxine AI and ML technology. This can perform object recognition within video frames, carry out speech-to-text translation, provide video and audio super-resolution, and add metadata to video and image files.
Minev says that discovered content can include events and people, and recognize who said what, when, and to whom. The events could be things like a goal scored or a penalty taken in a soccer game.
This is a huge step forward from manually tagging objects. It relies on sophisticated AI technology that can operate on documents, audio recordings, images and videos. Document content recognition is comparatively easier than looking into images and videos. The words “goal” or “penalty” can be simply located in text documents, but locating a goal in an image or video – which can view goalposts and goal keeper from different angles, and detect a football across the goal line – is several steps harder.
Quantum wants to automatically add metadata – annotations in effect – to documents, images and video recordings. This would enable users to search for things in potentially millions of files and objects, such as “find all penalties taken in Manchester United football matches in the last five years.”
It could aid manual searches too, by automatically summarizing file and object contents with a list of significant things or people in them.
CatDV would have this AI-generated metadata added to it, and then be used in media asset searching as an index through which to find content e.g. for reference or reuse in creating a broadcast news item.
There are separate AI technology areas here, such as natural language processing, voice recognition, and computer vision. We can envisage a suite of AI/ML capabilities being added to CatDV, perhaps with a vertical market focus. That would be needed because object recognition is situation-dependent. Object recognition in baseball, American football, rugby, soccer, cricket and tennis is different from object recognition in automobile events. What would be a ball in one of these could be a wheel in another.
Quantum could offer a CatDV media metadata discovery service that can check through media assets stored on premises or in the cloud – basically a content scanning operation.
The specific AI/ML services could be plug-ins – created by Quantum or third parties – and enable CatDV to look at video surveillance files or medical images, such as X-rays, CAT and MRI scans, using different ML models, and tag the stored items appropriately. The system could, for example, alert clinicians that a scan requires human inspection to verify a judgement about disease presence or absence.
The automated creation of such cross-content type metadata could well be the deciding factor in whether or not to buy a particular storage offering. In fact, the storage purchase could be entirely contingent on it. A media storage system covering on-premises and in-cloud file and object storage classes with automated tiering, AI-based content discovery and tagging could look much more attractive to a buyer than a bare bones file or object storage system – however low-cost or fast such a system might be.