VAST Data thinks that generative AI developments will lead to an “arms race” for real data as well as the ongoing one for GPUs for AI work. That’s data coming from humans and not generated by AI chatbots.
We were briefed by Andy Pernsteiner, VAST Field CTO, who was in in London for the Big Data event. Our discussion covered lots of ground, including VAST Data arrays being used to store data for training generative AI models, and its coming DataEngine compute layer. VAST Data is building a storage and AI/analytics stack, composed of VAST’s all-flash and single tier Universal Storage at the lowest level, then its data catalog with its proprietary transactional + analytics DataBase layered on that, and, above that, DataEngine software under development to operate on the data in the DataBase. Overall this a global DataSpace will provide a single namespace spanning VAST’s systems.
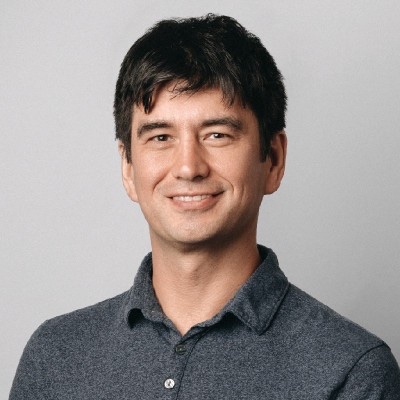
Pernsteiner said: “There’s going be a whole class of customers that don’t know what they’re doing in deep learning, who are going to want a solution that’s turnkey, that includes everything that they need. And so right now, obviously, we don’t have all of those things. We’re starting to think through what the next generation is. And that’s what the Data Engine is really all about; making it so that customers who don’t have the expertise of building out scalable compute infrastructures, can have it alongside their storage without having to go and layer it on themselves.”
This VAST AI and analytics system will ingest, as VAST Data CEO and founder Renen Hallak said: ”the entire data spectrum of natural data – unstructured and structured data types in the form of video, imagery, free text, instrument data,” which will be generated from all over the world and processed using real-time inference and constant, recursive AI model training.”
Hallak describes it as “natural” data, meaning generated by humans and human interactions, not created by AI chatbot-type entities.
Pernsteiner said: “If the content in the future is all generated using AI, this is training itself on its own data which can lead to a downward spiral.” He referred to this as model collapse and it occurs when a generative AI model is trained on data generated by AI and not human activities. This synthetic data is limited in scope and the AI model’s generates rules (weights) are limited as well, meaning the model gives more strength to likely events and patterns in the data, and less weight to unlikely ones, becoming unrealistic.
Model collapse was explained in a research paper by Canadian and UK computer science academics called, “The Curse of Recursion: Training on Generated Data Makes Models Forget”. It found that “use of model-generated content in training causes irreversible defects in the resulting models, where tails of the original content distribution disappear.”
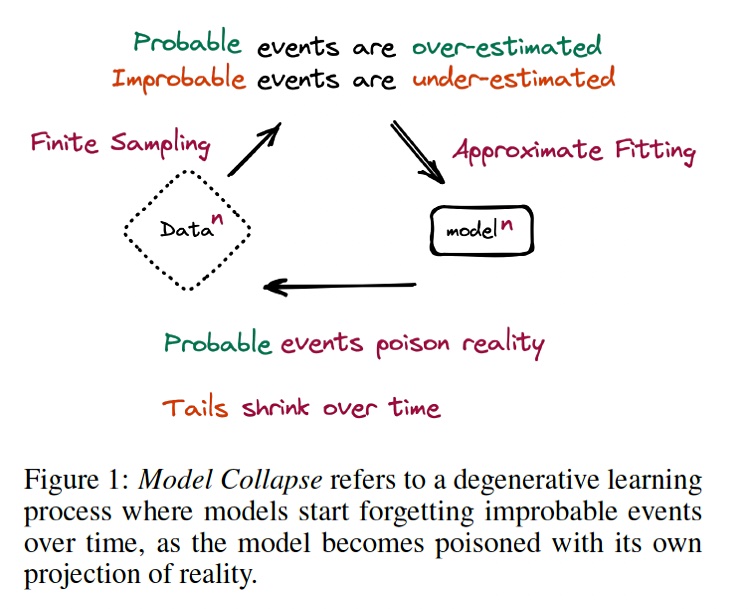
Large language models are trained on data scraped from the Web. Up until recently the overwhelming majority of that data has been created or generated by humans. Now increasing amounts of it are being produced by generative AI model such as ChatGPT instead. The researchers write in their paper: “If most future models’ training data is also scraped from the web, then they will inevitably come to train on data produced by their predecessors. In this paper, we investigate what happens when text produced, e.g. by a version of GPT, forms most of the training dataset of following models.”
What happens, the authors say, is that: “Models start forgetting improbable events over time, as the model becomes poisoned with its own projection of reality.”
Their paper’s abstract says: ”We demonstrate that [model collapse] has to be taken seriously if we are to sustain the benefits of training from large-scale data scraped from the web. Indeed, the value of data collected about genuine human interactions with systems will be increasingly valuable in the presence of content generated by LLMs in data crawled from the Internet.”
Pernsteiner said: “There’s a lot of debate in the community about models. Maybe it’s not necessarily a doomsday scenario. But it’s kind of a scary concept, when you can’t tell what’s real. And if a machine doesn’t know what’s real, what’s not real, how does it know to use it when it’s training a model or not? And so all the people developing models are trying to find ways to detect if the data is real or not. But how do you do that? How does a human, how do you know? How do I know that your blog post is written by you?”
The research authors write: ”the use of LLMs at scale to publish content on the internet will pollute the collection of data to train them: data about human interactions with LLMs will be increasingly valuable.”
Pernsteiner said: ”That’s why you see Meta, OpenAI, all of these forward thinking model creators, are acquiring data or having to buy it now.” They have to train their models on real data: “So real data will become more valuable to the point where any organisation that collects any data can monetize it.”
He asserts:” Any real data will become more and more valuable. … And if you’re an organization; it doesn’t really matter what kind of organisation you are, your data is valuable to somebody.”
He thinks organizations will need to build their own generative AI models because bought-in models that could have been trained on polluted data; model collapse-type data. He said: “If you’re an organization, and you want to use generative AI, you want to build a generative value practice and you want to make sure that your models are not tainted, then you have to build your own.”
This means, he reckons: “Organizations who develop their own models, who have things of value; they can start selling those as data products as well.” Also: “If you’re a big enterprise, you might be able to sell your model. And that might be where money is made in the future; selling models.”
There’s an audit angle to this as well. In regulated industries the authorities may need an AI-made decision, that led to an accident or suspect event, examined. Pernsteiner said: “Now I’ve got to understand the products a little bit more. The data products are not going to be limited to structured data. It’s going to include things like the model, the source data, the annotated data, all of the labels, any vector databases that you have that are associated with it … Show me all the lineage. Show me the model. Show me the training data you use. Show me the code you use. And it has to be immutable.”
Pernsteiner thinks that: “All these things are interesting because it makes it so that regular companies, if they want to survive; they can’t just rely on selling the products that they’ve been selling in the past. They’re going to sell data, they’re going to sell models. They want to train their own models, they need a lot of infrastructure. Maybe not exabytes of data. But they need all their data, they might need to buy data from other people. It’s not just an arms race for infrastructure, it’s an arms race for data.”