HPC storage supplier Panasas is working with MLCommons on how best to measure machine learning (ML) storage performance, develop an ML storage benchmark, and help develop a next generation of storage systems for ML.
MLCommons is an open engineering consortium which set up the MLPerf benchmark in 2018. This is a set of industry metrics to measure machine learning performance and promote transparency of machine learning techniques. There are more than 50 founding partners – including global technology providers, academics and researchers. MLCommons says it’s focused on collaborative engineering work that builds tools for the entire machine learning industry through benchmarks and metrics, public datasets and best practices. It promotes widespread ML adoption and democratization through benchmarks, large-scale public datasets, and best practices.
David Kanter, founder and executive director of MLCommons, provided a supportive quote: “The end goal of the MLPerf Storage working group is to create a storage benchmark for the full ML pipeline which is compatible with diverse software frameworks and hardware accelerators.”
Panasas said it approached MLCommons to discuss the storage challenge in the ETL (extract, transform, and load) process and its impact on the overall performance of the ML pipeline. At that point MLCommons had been in the early stages of forming an MLPerf Storage working group to develop a storage benchmark that evaluates performance for ML workloads including data ingestion, training, and inference phases.
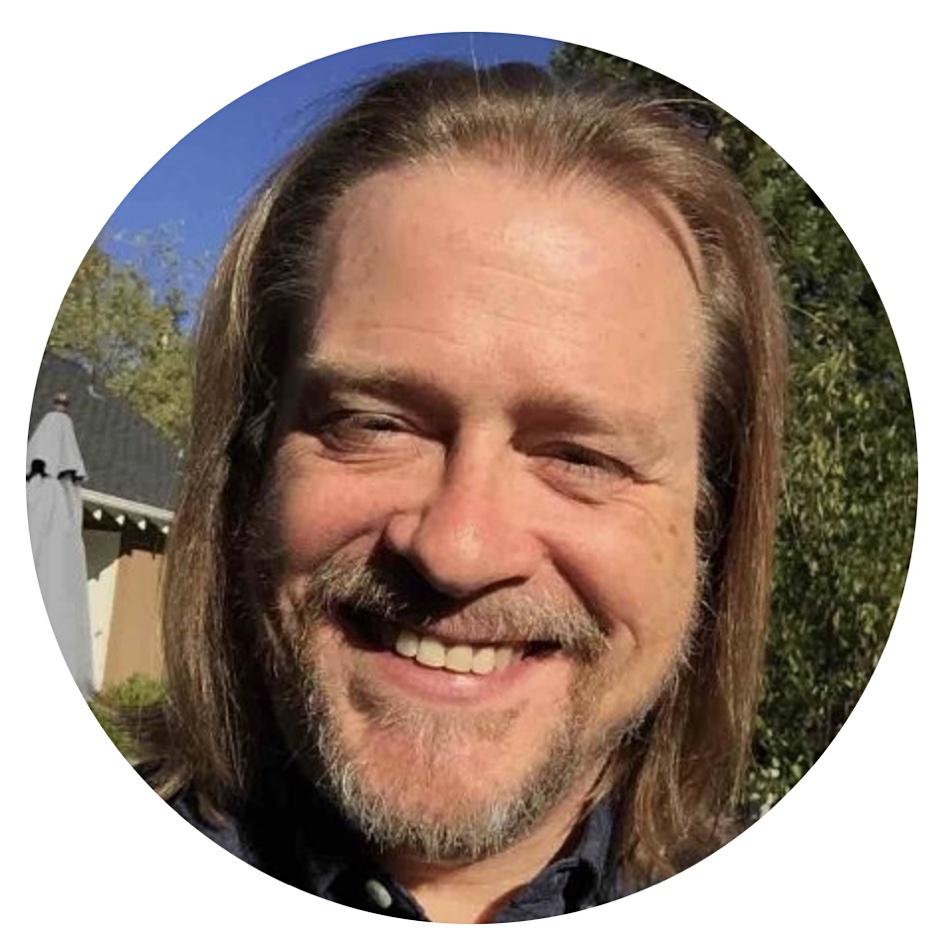
MLCommons invited Panasas to attend the foundational meetings, after which Curtis Anderson, a Panasas software architect, was named co-chair of the MLPerf storage working group. That was actually back in March – the announcement took a while to come out. He will be working with the group to define standards for evaluating the performance of storage subsystems that feed AI/ML environments and develop a storage benchmark that evaluates performance for ML workloads including data ingestion, training, and inference phases.
The group’s deliverables are:
- Storage access traces for representative ML applications, from the applications’ perspective – initial targets are Vision, NLP, and Recommenders (short-term goal);
- Storage benchmark rules for:
- Data ingestion phase (medium-term goal);
- Training phase (short-term goal);
- Inference phase (long-term goal);
- Full ML pipeline (long-term goal);
- Flexible generator of datasets:
- Synthetic workload generator based on analysis of I/O in real ML traces, which is aware of compute think-time (short-term goal);
- Trace replayer that scales the workload size (long-term goal);
- User-friendly testing harness that is easy to deploy with different storage systems (medium-term goal).
Kanter said “I’d like to thank Panasas for contributing their extensive storage knowledge, and Curtis specifically for the leadership he is providing as a co-chair of this working group.”
There are two other co-chairs: Oana Balmau, assistant professor in the School of Computer Science at McGill University, and Johnu George, a staff engineer at Nutanix. We don’t have access to the working group membership list, but we have requested it. Having three co-chairs suggests it is quite large. Any storage supplier looking to feed data to machine learning applications could well be interested in joining it. For example, Dell EMC, DDN, HPE, IBM, Infinidat, Intel (DAOS), MinIO, NetApp, Pure Storage, StorONE, VAST Data and Weka, plus the main public cloud providers.