IBM says machine learning could be accelerated by up to a thousand times by using analog chips based on Phase-Change Memory.
Phase-Change Memory is based on a chalcogenide glass material which changes its phase from crystalline to amorphous and back again when suitable electrical currents are applied. Each phase has a differing resistance level, which is stable until the phase is changed. The two resistance levels are the basis for binary one or zero values.
PCM devices are non-volatile and access latency is at DRAM levels, making them an example of storage-class memory. The Intel-Micron 3D XPoint technology is based on PCM.
An IBM blog reveal the company is setting up a research centre to develop next-generation AI hardware and look at nanotechnology. Technoloy partners includeNew York Staye, the SUNY Polytechnic Institute, Samsung, Mellanox, and Synopsis.
New processing hardware
The blogger, Mukesh Khare, VP semiconductor and AI hardware at IBM Research, says current machine learning limitations can be overcome by using new processing hardware such as;
- Digital AI Cores and approximate computing
- In-memory computing with analog cores
- Analog cores with optimised materials
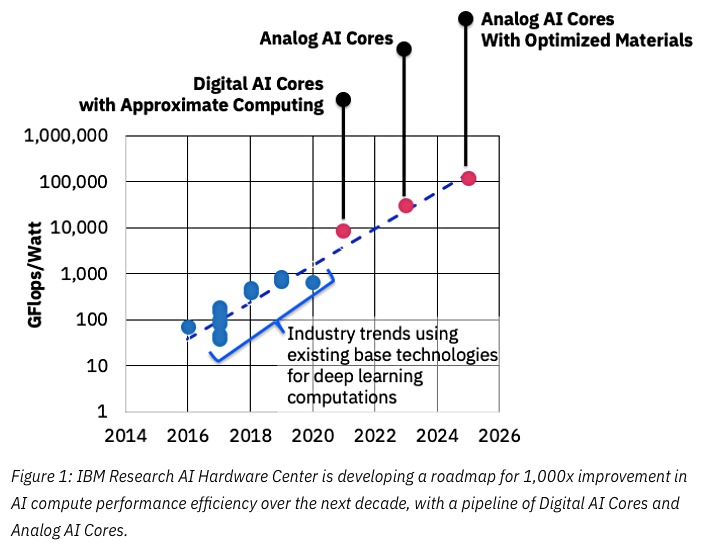
He mentions mapping Deep Neural Networks (DNN) to analog cross-point arrays (Analog AI cores). These have non-volatile memory materials at the array crosspoints to store weights.
Numerical values in DNN calculations are weighted to improve the accuracy of decisions in the course of training.
These factors can be arrived at directly with a crosspoint PCM array without needing host server CPU intervention so providing in-memory computing, with no need for data movement. This is an analog array, in contrast with digital counterpart such asIntel XPoint SSD or DIMM.
The PCM cells record synaptic weights along an 8-step gradient between the amorphous and crystalline states. The conductance or resistance of each of the steps can be altered with electrical pulses. These 8 steps provide 8-bit precision in the DNN calculations.
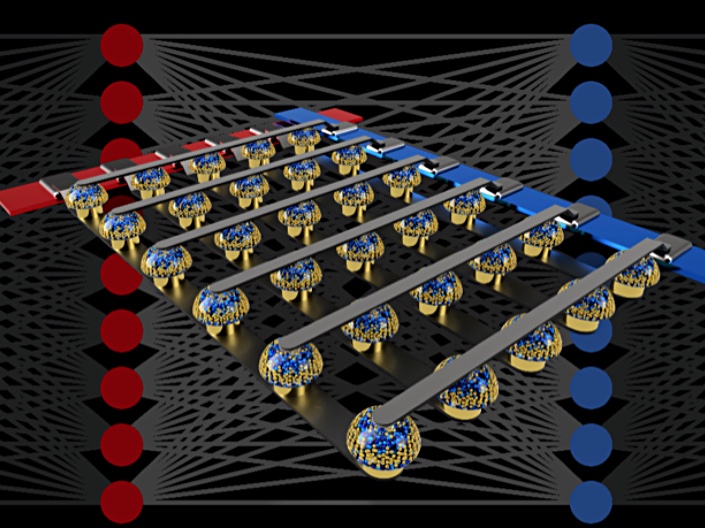
Computation inside the analog memory chip
The IBM research paper states:
“Analog non-volatile memories (NVM) can efficiently accelerate the “backpropagation” algorithm at the heart of many recent AI advances. These memories allow the “multiply-accumulate” operations used throughout these algorithms to be parallelized in the analog domain, at the location of weight data, using underlying physics.
“Instead of large circuits to multiply and add digital numbers together, we simply pass a small current through a resistor into a wire, and then connect many such wires together to let the currents build up. This lets us perform many calculations at the same time, rather than one after the other. And instead of shipping digital data on long journeys between digital memory chips and processing chips, we can perform all the computation inside the analog memory chip.”
Check out an IBM AI Research blog page for more articles exploring these ideas.