Interview: GridGain software provides a way for clustered servers to share memory and therefore run apps needing more memory than that supported by a single server.
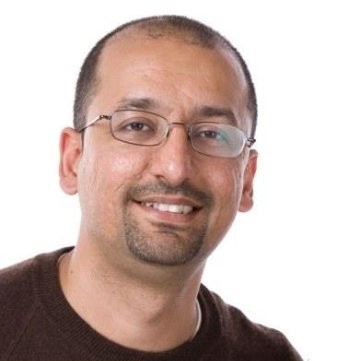
Lalit Ahuja, GridGain CTO, reckons that its memory capacity enhancing tech makes GridGain a natural partner for AI work.
VC-backed GridGain was started in 2007 and has taken in $52.1 million in funding, according to Crunchbase. The most recent round was a $13.5 million Series C in 2021. It has many customers, with 170 listed on its website.
GridGain software provides a distributed memory space atop a cluster or grid of x86 servers with a massively parallel architecture. The GridGain-developed software was donated to the Apache Foundation, becoming Apache Ignite, an open source distributed data management system using server memory as a combined storage and processing in-memory tier, backed up by an SSD/HDD tier.
Data is stored using key-value pairs and distributed across the cluster. The software can be deployed on premises, on x86 servers or IBM z/OS mainframes, and in the Azure, AWS, and Google clouds. GridGain supports Optane storage-class memory and also GPUs via its HydraDragon hybrid transactional/analytical storage engine.
GridGain’s focus has been for several years on high-performance, real-time analysis of enterprise data held in data lakes and semi-structured data in non-relational/NoSQL databases, and making them far less IO-bound.
The GenAI surge in interest means that it views large language model (LLM) processing based on vector embeddings as another workload for its memory grid. We asked Ahuja some questions about GridGain and Gen AI.
Blocks & Files: What contribution can GridGain technology make to AI training?
Lalit Ahuja: GridGain is a unified real-time data platform that combines streaming data processing with historical contextual data and the execution of complex AI workloads into a single platform. With its unique distributed, in-memory data processing architecture, GridGain can extract features out of incoming streaming events, combine them with historical offline features also held in GridGain, and execute continuous training of AI models, thereby training and making newly trained models available for execution faster and on an ongoing basis.
A great example of this would be healthcare claims fraud prevention, where new ways of fraud keep coming up to circumvent the claims processing checks and balances and one has to continuously update their fraud detection models to stay ahead of the bad actors.
Blocks & Files: What contribution can GridGain technology make to AI inferencing?
Lalit Ahuja: The key to accurate and reliable inference is the availability of all the necessary data, and the latest, most current version of that data. By combining event stream processing with transactional and historical data, GridGain can enable real-time AI inferencing. GridGain can easily be deployed as a low-latency data hub over hundreds of data sources of all types (structured or unstructured, on-prem or in the cloud), and when combined with GridGain’s event stream and transactional data processing capabilities, ensures that the AI model has the complete data it needs for inferencing.
A great use case for this real-time AI inferencing capability would be in the capital markets side of financial services, where financial risk analysis decisions have to be made and the decision depends on the latest price of the different assets from various asset classes (equities, commodities, derivatives, currencies, etc.) in scope. If the latest price for these assets is not used, then any risk analysis, hedging strategies or financial portfolio structuring decisions will be flawed.
Blocks & Files: How does GridGain view CXL 1 and 2.0?
Lalit Ahuja: It is something we are cautiously optimistic about and are closely watching. We are also actively speaking with our customers to gauge what their interest level is in this technology.
Blocks & Files: What will CXL 3.0 bring to the party for GridGain?
Lalit Ahuja: Specifically for CXL 3.0, the distributed memory resource sharing capabilities and persistent memory hold a lot of promise but, again, a lot of this depends on what the adoption rate of such a technology is among our customer base.
Blocks & Files: Can GridGain’s memory space encompass GPUs and their HBM? Is the situation the same for Nvidia, Intel and AMD GOPUS/Accelerators? What about storage-class memories such as MRAM and ReRAM? Are these anything other than niche use cases?
Lalit Ahuja: GridGain is experimenting with partners like Nvidia, Intel, and SK Hynix in these areas.
Comment
Ahuja talks about GenAI training in the context of refining in-use large language models and not in the context of training such models in the first place. That currently requires large numbers of GPUs with their high-bandwidth memory. This is an area where GridGain is talking to the main suppliers such as Nvidia and Intel, and also HBM supplier SK hynix.
We understand that, as Nvidia has high speed chip-to-chip interconnects, delivering 900 GBps of total bandwidth in its Hopper superchip, which is seven times faster than the PCIe gen 5 bus, it has less need for a single HBM3e memory space than if it were stuck at the PCIe 5 level.
As Intel is no longer developing Optane, the use of either MRAM or RERAM as a substitute storage-class memory is going to depend, B&F thinks, on enterprise adoption of these technologies.