Panmnesia, a technology firm based in South Korea, unveiled its pooled CXL memory system at Flash Memory Summit (FMS) 2023. In a live demonstration, the system showcased performance metrics more than three times faster than an RDMA-based system while running a Meta recommendation application.
The CXL technology behind this was developed in partnership with the Korea Advanced Institute of Science & Technology (KAIST) located in Daejeon. Panmnesia has crafted a comprehensive CXL framework that includes CXL CPU, Switch, and Memory expander modules. This framework possesses 6TB of DIMM-based CXL memory. The company’s strategy involves marketing its CXL hardware and software intellectual property products to CXL system developers and manufacturers.
Dr Myoungsoo Jung, Panmnesia CEO, said: “We’re excited to introduce our innovative multi-terabyte full-system CXL framework at this year’s Flash Memory Summit. Our commitment to pioneering cutting-edge solutions is demonstrated through our CXL IP, which we believe will significantly enhance memory and storage capabilities within data centers.”
The demonstrated system has a capacity three times larger than the 2TB Samsung/MemVerge-based pooled memory system also displayed at FMS 2023.
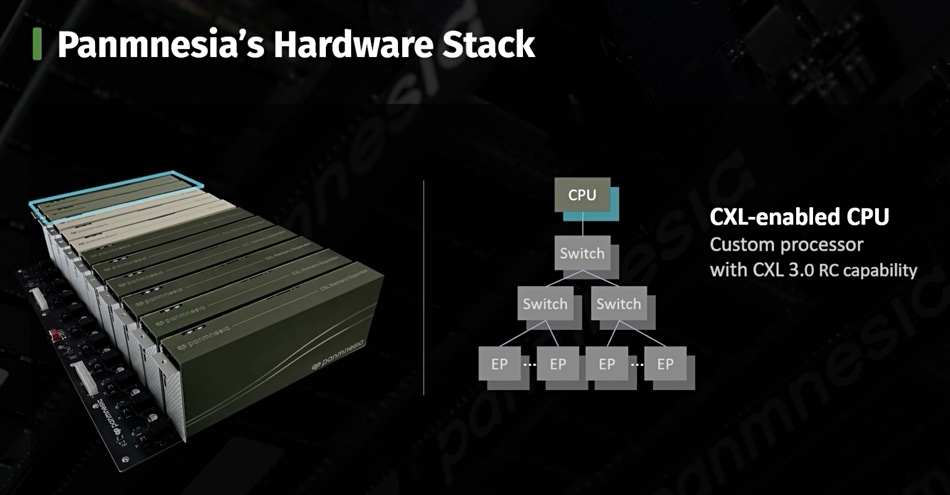
The Panmnesia framework system chassis has two CXL CPU modules, highlighted in the diagram above, three lighter colored CXL switch modules, and six 1TB memory modules or CXL endpoint controllers, forming a single DIMM pool.
On the software side, the system runs on Linux and consists of CXL hardware device drivers, a virtual machine subsystem, and a CXL-optimized user application. Interestingly, the virtual machine software component creates a CPU-less NUMA node within the memory space.
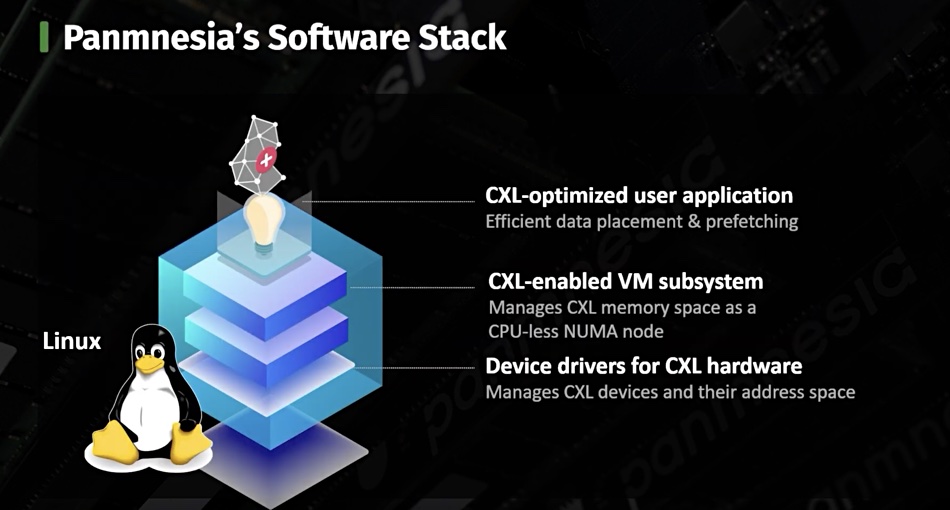
A video slide deck shows the performance of this CXL framework system in a movie recommendation application akin to Meta’s recommendation application. This is again compared to an RDMA-based alternative where the servers involved had no added external memory.
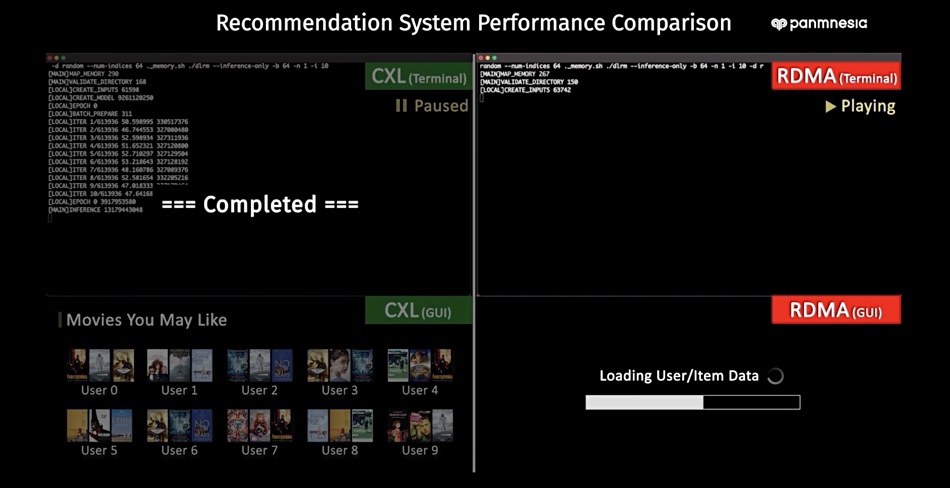
The video starts with the loading of user and item data (during Tensor initialization) and subsequently used a machine learning model for movie suggestions. The Panmnesia system completed the task 3.32 times faster than its RDMA counterpart.
An additional benefit of Panmnesia’s system is its modularity; DIMMs within memory modules can be substituted, allowing for a scaling up of memory capacity using larger-capacity DIMMs rather than incorporating additional memory modules.