Startup Lightbits is claiming its software-defined block storage is better than competing products because it’s built for high performance and scale.
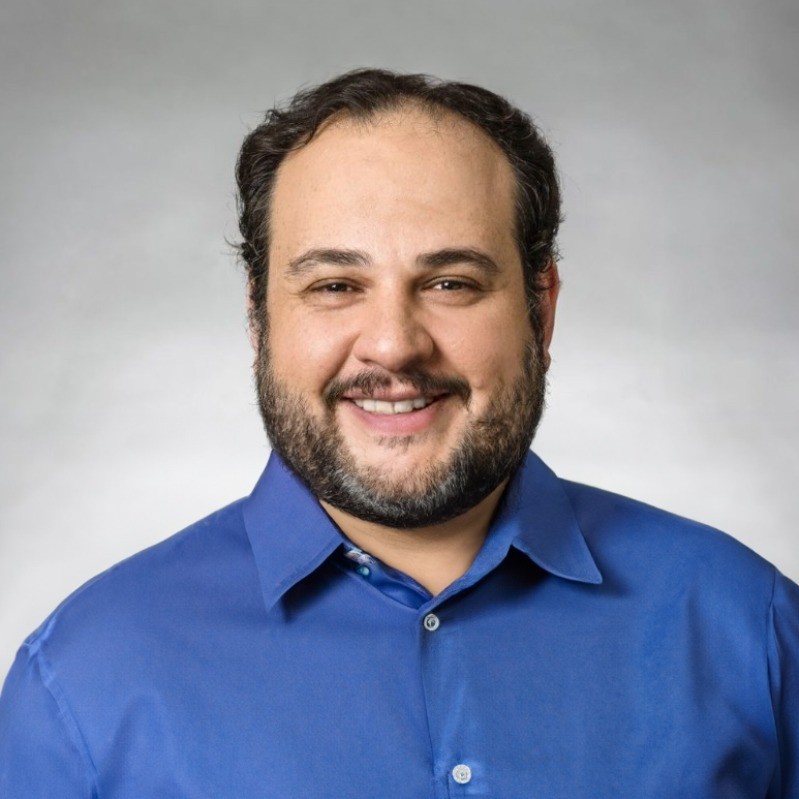
This applies particularly to the training and inferencing stages of the AI pipeline, CTO Abel Gordon claimed in a briefing with Blocks&Files.
Lightbits block storage software runs in the AWS and Azure clouds, where it uses ephemeral storage instances to make its block storage faster and – we’re told – more affordable than both AWS and Azure’s own block storage instances. It can also run in private clouds on-premises. The Lightbits software creates a public or private cloud SAN by clustering virtual machines connected by NVMe over TCP, and can deliver up to 1 million IOPS per volume with consistent latency down to 190 microseconds. Increasing the cluster size boosts storage performance linearly.
Gordon told us Lightbits disaggregated software is good for AI training workloads because you don’t get stranded storage, excess data copies, or vulnerable AI servers, as a slide illustrates:
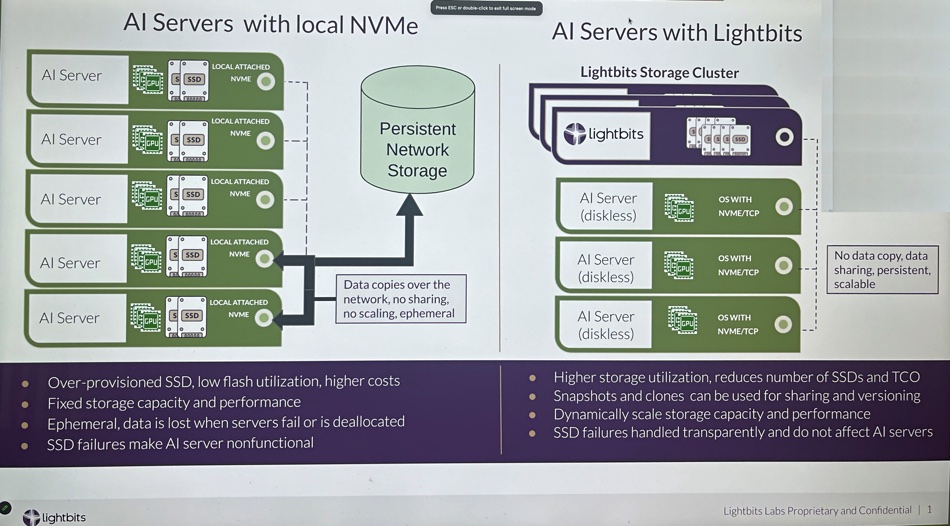
He asked readers to imagine a traditional cluster approach with AI servers having their own directly attached NVMe flash drives. He said this provides no sharing, needs data copied over the network, has no scaling, and AI servers go offline if their SSD fails. A disaggregated Lightbits storage cluster shares storage between drive-less AI servers and can scale with Lightbits saving the data to persistent storage. Moreover, drive failures do not cripple an AI server.
Gordon added that training runs, which can last for several hours or more, require the application to be regularly checkpointed with its state saved to storage. If the training run fails for some reason, it can be restarted from the last checkpoint instead of starting from the beginning, he said. This can save a lot of time when recovering from failures.
The faster the checkpoint data is written to storage, the less overhead time is required. Similarly, quicker restoration of checkpoint states allows for faster restarts.
Lightbits block storage is also well suited for vector database and retrieval-augmented generation (RAG) work in AI inferencing:
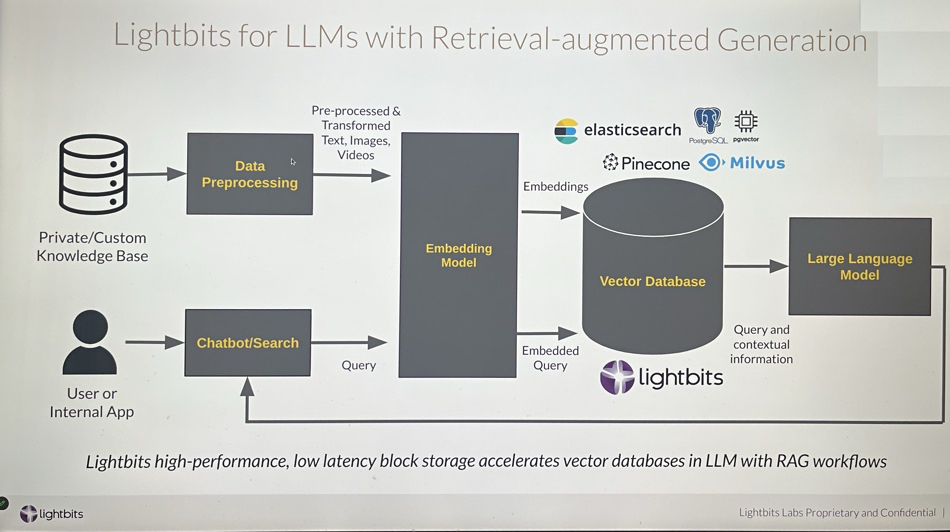
A RAG AI pipeline starts with an organization’s own data – text, images, videos, audio recordings, etc. – which is transformed into vector embeddings via an embedding model. The output embeddings are stored in a vector database from a supplier such as Pinecone or Milvus, and this, Gordon said, can use Lightbits block storage.
Over time, he added, images will be used more and the size of the vector database will grow. As Lightbits provides scalable block storage, this is no problem.
The Lightbits software’s low latency and high performance helps speed large language model (LLM) conversational query responses.
The Crusoe AI Cloud uses Lightbits storage. Mike McDonald, Crusoe product manager, said of this: “Lightbits on Micron SSDs blew everything else out of the water, with consistently higher throughput, IOPS, and lower latency across the board. We were impressed with the performance and consistency of Lightbits.”